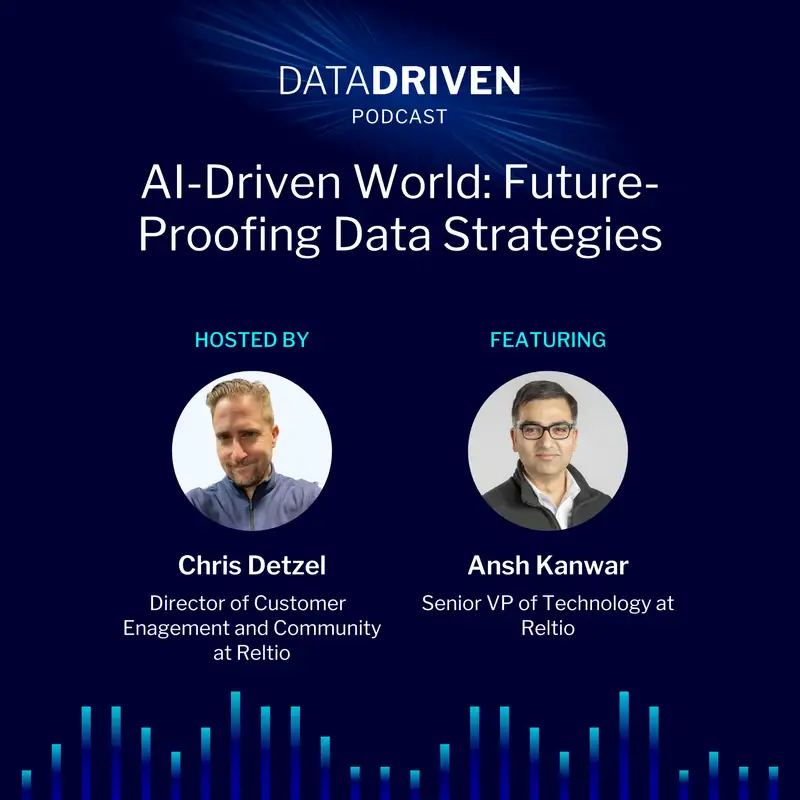
AI-Driven World: Future-Proofing Data Strategies
Welcome to another data driven podcast. I'm Chris Detzel. And today, we have Ansh Kanwar. Ansh, how are you today?
Ansh:Hey, everybody. How are how's it going? How's it going, Chris?
Chris:I'm doing well, man. Thanks. Just got done running a half marathon this weekend. Did you do anything fun this weekend? Or
Ansh:Nothing nothing as exciting as that. But I I I do hear that you didn't even break a sweat doing that.
Chris:Oh, I did. So, I always break a sweat during any kind of runs, but, well, you
Ansh:know look great.
Chris:Yeah. And I appreciate that. And, you know, I appreciate you coming on again. So this is, you're you're not a guest anymore. You're just a regular.
Chris:So appreciate that.
Ansh:No. I'm I'm the guy who hangs out in the back. Just put a free slot.
Chris:I just asked the questions. You have to answer them. You gotta be the smart one. So, today, you know, something that is super hot today is is around AI. And and specifically, I wanted to talk a little bit about concerns around data security and privacy, which is becoming, you know, a bigger consideration for organizations as they integrate more AI tools into their arsenal, more specifically around data management.
Chris:So I wanted you to kinda give us the lay of the land. Tell us a little bit about some of the AI related data security privacy issues that have popped up here in 2023.
Ansh:Yeah. Absolutely. So, it's it's been the the year that AI finally burst upon the scene, right, as as a mainstream conversation and thanks to chat gpt. But AI related research has been sort of trending towards that now for the last 10 years. Right?
Ansh:The the AI winter of the late eighties, nineties that that that sort of got, pushed away as soon as we learned that by using big data and very large amounts of data, we could do enough pattern matching to then create at least the illusion of intelligence and really with all the different schemes that now culminate, with large language models, it really felt like AI is now accessible to all of us. Right? Technologies. Art. And, you know, it's, folks like, my mother who's 75 about turning 75.
Ansh:She's asking me questions about about AI, in her in her own way, and, it's just just been fantastic to see all of that. But at the same time, within these last 10 years or so, the law hasn't really kept up with the innovative uses of of this technology. And so we've gotten ahead the the the the the cognitive ability, if you will, artificial artificial intelligence has gotten ahead of where we are as a society. And that started really showing up in 2023. Right?
Ansh:So while the main news was focused on chat gpt and trends, here's some examples that we dug up on what else was happening in this in the the privacy and and security space. So in Jan 2023, Clearview AI, facial recognition company, they were fined 20,000,000 by the UK information commissioner's office, and that was for, facial recognition, sort of unauthorized facial recognition, and they were scraping and sort of keeping, images around without permission. Deepfakes deepfakes have been growing in the depth of the fakeness, if you will. Right? So the they're they're starting to have the implication they they're starting to get reactions from people, and as we just don't know any better.
Ansh:You know, when the Ukraine, Russia conflict, there was a of a fake video that that was started circulating that had the president of Ukraine making statements that caused a lot of panic and confusion. As expected, AI is being used to weaponize the cat and security breaches and security tools, right, to to try to protect against those breaches. And an example of that was, also in 2023, T Mobile announced a big hack, where hackers have stolen personal information. This was, like, 37,000,000 subscribers worth of information. And they that hackers gained access through, a vulnerability that was in an API that was being used to power an AI powered chatbot.
Ansh:Right? So this is this is, an associated example, a side example, if you will, of a vulnerability. But rest assured that there is more and more in terms of vulnerabilities that are shared publicly. Some of them are now being discovered using machine learning tools, and some of them are being powered by ML algorithms.
Chris:By the way, I'm not surprised about all of that, you know, and something that I've been talking to some, folks around is there's this one program that allows you to create, you know, voices and, you know, you can type in like conversation or videos. Right. You know, on like my voice. So I could do Chris Detzel, set these things and it has my exact voice and I can push it out there into the ether. Right?
Chris:And you can do it in a video. You can do I mean, it it I've I've seen it and I've kind of played with it. I could put it, I could put this conversation into, into French. I could put it into, you know, different kind of languages. Right.
Chris:Right. That's right. There's ways to do that today. And so, you know, there's, there's lots of opportunity to take advantage of those things. Oh, you know, and and and and and make those things fake because it's easy to do now.
Chris:Like, it's so very easy.
Ansh:The the and then right there, you highlighted the immense promise and productivity gain of these technologies. Right? That's why we're chasing them. There's just really nothing there's been nothing like them in the past. That's right.
Ansh:Time, the side effects, the possible sort of nefarious uses of these technologies also exist. I have to mention that on a plane recently, I watched the latest Mission Impossible movie. Mhmm. And they did a really good job of of of sort of using this, artificial voice creation to to hijack a very interesting scenario. I'll I'll leave it at that, but it's very Yeah.
Ansh:Good to go watch. If you if you wanna take it to the other extreme of action movies, there's some plot points happening because of it. But but but back to back to our list. Right? So, algorithmic biases continue to show up in many different, many different ways, right, in terms of decisions around hiring, decisions around, you know, there was, like, in Feb 2023, the at Amazon, there was, I think there was a court case about this where, hiring processes were biased against women, and that turned out to be based in, based in the model that was being used to filter out the applications.
Ansh:And then finally, I think it's it's, it's more of a societal discussion where there is increasingly this lack of transparency on decisions that are being made by, by algorithms and the general public is concerned. I think the latest, survey by Pew Research said 72% of Americans are concerned about the lack of transparency in how companies use their data. Right? And this doesn't even have to do with AI machine learning. It's just simply a privacy concern, but but that data inevitably in the future will be used by AI models.
Ansh:And so these are intertwined concerns. Yeah. Yeah.
Chris:I appreciate that overview. That's that's extremely helpful. Can you talk a little bit or explain to some of our listeners on how data unification and management tools, such as, you know, master data management intersect with data security and privacy in the context of, like, AI, integrations?
Ansh:Well, we'll go there, but but let's start with how these tools work even without AI. Right? And, the the the problems they solve, essentially boil down to the fact that data valuable data, such as data about customers, data about suppliers, it's fragmented. It's distributed into so many silos. Right?
Ansh:And this fragmentation happens for all, appropriate business reasons. For example, if an enterprise acquires another company or decides to spin out a business unit, or just departmental uses of this customer data. Let's just use customer data as an example for this, this conversation. Yeah? So there's there's some of that in ecommerce systems.
Ansh:The customer support folks need to need some copy or variant of that data. You know, IT systems have that data and so on. So, ultimately, you have all of this fragmented data. And what, a modern data unification system does is really able to bring that data back together to give you this trusted view of what your customer who your customer is. Right?
Ansh:And so that's either called data unification or data mastering as a process. And what's happening behind the scenes is to to to do this, every unique piece of data or every unique entity, if you will, is being provided with an identifier. Right? And this identifier, in a way, it it points to or or not in a way, but it points to all the information about that individual that's available to that enterprise as first party information. Right?
Ansh:Yeah. And so this has some interesting implications in terms of privacy because guess what? Now you're referring to an individual through an ID instead of all the personally identifying identifiable information that was sort of spread out through all these systems. Right? So keep that keep that idea in mind.
Ansh:The other part of what these systems do is is really help you have confidence in that customer data. So it's it's it's one thing to, suspect that you have a 100000 customers, but you may have that data from different systems, as the sources. And so you, you know, it's another thing to be able to say with confidence, with a very high level of confidence that you have 990,000 customers. Right? 991,000 customers or so on.
Ansh:Right? And so it's a it's a question of precision, and this question of precision becomes much more important, when there is a high stakes transaction that's that's that's associated with, with those customer profiles, a very large volume of transactions. Now as an example, take loyalty programs. Right? So a good loyalty program, it's predicated on the understanding of, on the one hand, the preferences and desires of your customer.
Ansh:On the other hand, you also want to as as as, you know, as part of personalizing your offers to your customer, you want to be able to have enough data that allows you to personalize that offer. Right? So that's a perfect example where having the right underlying technology allows you to balance the need for, for for for the the personalization versus privacy conundrum. You can think of, like, a global franchisee model for a for a quick food or fast food type of establishment. Right?
Ansh:In that scenario, what's happening is the data about your customers is now fragmented because of the franchisee model. You have customers who may be, buying your product in multiple countries. Right? So having flexible foundation where there is trust in the data and you're able to, operate with confidence knowing who you're dealing with as a customer and you're respecting their their privacy, as well as, creating offers from them regardless of where they are, regardless of where they are. That's that's a very, very tough but very critical, balance to be able to strike for our customers.
Ansh:Does that resonate with you, Chris? That that sort of situation?
Chris:Yeah. I think so. I think that's a a good for you. It's a good answer to that piece of it. But when you start thinking about the I AI type of pieces, you know, you know, from a data security standpoint or privacy, anything around that piece?
Ansh:Yeah. Okay. So now let's take this and apply this to the AI integration, process. Right? Yeah.
Ansh:So there's really 2 ways in which, the the the data model interacts with the AI model, if you will. Right? The first way is that you use this data to train your AI models. And the direction, you know, that that that training depends on the data, the model data being accurate, that model data not having enough biases. And essentially being in a state where you know where that data is coming from.
Ansh:And if there are defects that creep in over time, you know, where what is the source of those defects? Right? So, ultimately, these models are trying to extract, value or subtract trying to extract signal from sometimes very sparse data about your broad customer base. Right? So how how how do you get the the underlying data to be in a place where you can you can extract that signal?
Ansh:Right? So that's kind of the one direction. The other direction is if you start with the model, and then and have it act on your data. So if you're trying to run a model to segment your customer base, for example, by, by the propensity to buy a certain kind of object. Well, what you're trying to segment has to be in a decent state so that the segmented data, when activated, can actually help you meet your business goals so that the their growth related or retention related growth.
Ansh:Right? So think of think of those two dimensions. And both of these dimensions, either building a model or using a model, they really depend on the accuracy and the effort, the the the the trustworthiness of the data that's really underlying any of these operations.
Chris:That's good. So what are the primary risks and challenges associated with data privacy and AI systems? And, you know, how do you how does effective data management address these issues?
Ansh:Right. So it's a fairly broad question, and we highlighted some of the some of the areas where these risks were present and realized just in 2023. Right? At the highest level, the way I think about it is that, you know, imagine you are running an enterprise, enterprise, and I give you a magical black box and say, you can increase your growth 5% or 6%, whatever, over over your whatever your baseline is. But you can't really see what the you know, you can't really it it's inscrutable what the box is doing.
Ansh:That is the definition of black box. At the same time, everybody else have that black box as well, and it just has this word, like, accelerate, stamped over it. Mhmm. It's it's irresistible, and therefore, you are going to invest in it. You are going to, you know, adopt it within your operating model.
Ansh:Right? But it comes with this perverse incentive that this this this black box makes decisions, and it makes decisions, just just it's right just enough that there's a perverse incentive to pull the human out of the loop of that decision making. Right? What you have is something that's making decisions faster and faster So how do you how do you manage this this technology this this technology so that it stays on the rails and and is is governable? It is it is now producing results that are, you know, in line with the, the the legal and ethical, boundaries that you have set up for your business.
Ansh:Right? So my favorite story is is is a very simple one. It's about a seating chart. So, we decided to have a party, and, this was at the company. And we had 50 people that we wanted to seat around, 8 tables, I believe.
Ansh:Mhmm. And so somebody decided to pass this, this this this this action to an intern. The intern stuck that whole list of people into chat gpt and said, please build me a seating chart.
Chris:And Chat GPT
Ansh:did a beautiful job of building the seating chart. Right? Here's the here's the proof of people. Off you go. And, it's it's something was off because when it came back, there were 51 people instead of 50 people who were at these tables.
Ansh:And we had to go through name by name to figure out who was not an employee at the company. Right? Yeah. And so, you know, some some of this is related to hallucinations and and the fact that, you know, hallucinations are a fact of life right now. And, yes, they will go away over time, but it does illustrate this this very interesting, relationship between data and AI models.
Ansh:Right? Because that fifty first name was detected only because we we looked at the data and really inspected that output for its accuracy. Right? If we also had some sort of a reference data set in our mind, which said, what, you know, what are the what is the correct list of employees at the company? Yeah.
Ansh:Right? So those two things, together helped us detect this issue that came out of very simple ask to a large language model. Right?
Chris:You know, it's funny because just that one little thing, I had lots of questions around the seating chart. Right? Did they copy and paste one more name? You know, did it actually hallucinate? Just think about thousands of pound, thousands of names.
Chris:Copy and pasting that. You know? Yep.
Ansh:And and you wouldn't catch it. Right? At at enough scale, it'd be and and, the rate of of change in low latency system. That that'd be pretty pretty the catch. So I I think that's that's the crux of the matter, right, where, you know, we thought previously about this asymmetric power around facial recognition that companies with this technology have over society.
Ansh:Biases, you know, I'm reminded of this, ProPublica article I I, read it in 2018, I believe. Right? And that had to do with deciding on parole outcomes and how that particular algorithm that was being used with commercial algorithm had significant biases, you know, especially against African American people. We we talked about this AI powered arms race. We talked about the societal impacts, such as, you know, deep fakes and so on.
Ansh:And in a way, you can say all of this is sort of early technology around AI, especially large language models. But it is so deeply intertwined with these notions of privacy and individuality and fairness that, you know, it I I think this is hardly the first chapter in this book that sort of, solves for these problems in a way that our society can can absorb.
Chris:For sure. It's just the beginning. There's no doubt. How do you, how does, data unification strategies help organizations comply with data privacy regulations like, you know, GDPR and integrating when integrating AI?
Ansh:Yeah. Yeah. So, I'll answer 2 parts. Right? The the first part is GDPR, and, you know, think about the scenario where you have, customer data that's spread amongst many, many, many systems.
Ansh:Right? Mhmm. Now imagine a GDPR, forget me type of request that comes in. You would have to hunt and pack every one of those systems and ensure that that customer's data is no longer in your system or is is is deleted. Now fast forward, if you've implemented a data unification system, a system that's sort of connected to all of these source systems, ideally, what has happened is you are you now have a single trusted record about that customer in your data unification system.
Ansh:And you know exactly where the data about this customer has has been sourced from, where that has come from. So now you have the sing single stop shop, if you will, where you can go in and mark that data as deleted and then propagate it back towards all of the source systems and ensure that your GDPR type of requests are are honored. Right? The other angle is, where's your customer data located? Right?
Ansh:It's it's very important increasingly to make sure that geo geographical residency, of the data is mapped to the geographical residency of the citizen. Right? So, in China, for example, the the, MLPS, laws are are being enforced more and more, and that means that, PII and PHI around, Chinese, citizens really needs to be resident in China. You know, with, GDPR, there is a requirement for European data to be either in European mainland and and there's no extensibility to the United States, but very similar provisions are being passed all over the world. So data residency is also a very important, angle to to think through.
Ansh:Right? Mhmm. But how does this this apply to AI? Well, you fast forward and, the European Union is at the forefront of enacting laws that are similar to GDPR, but, really, you know, they're they're thinking in terms of public good. And I think they just, you shared an article with me this morning, and and they just released the, European Union AI Act of 2023.
Ansh:And I would like to read actually the some of the snippets of of of of that act because it is a window into how a lot of this legislation is gonna go in the future. So, they agreed on safeguards for general purpose artificial intelligence. They limited the use of biometric identification systems by law enforcement into, bans on scoring and AI to manipulate, and exploit vulnerabilities. So this is the security angles that are coming back in. Right?
Ansh:The right of consumers to launch complaints, they're preserving that right and to receive meaningful explanation. And they're backing that up with fines, like ranging from €35,000,000 all the way to 7% of global turnover.
Chris:Oh, €35,000,000,000. Oh, €35,000,000,000 also.
Ansh:I'm sorry.
Chris:It's it's a lot more than €35. That's all I'm saying. But yes.
Ansh:€5,000,000. Yeah. Right. Or 7% of global turnover. Right?
Ansh:So Yeah. And they did a good job, I think, of of sort of, classifying high risk AI systems. So anything that has to do with, you know, insurance and banking sectors or, systems that can influence the outcome of elections, for example. Right? All those are classified as high risk systems, and then a lot a number of, the the number of, sort of escalated or enhanced, level of scrutiny is is applied to these systems.
Ansh:So I I think this is the first, first shot by the European Union, and a lot of other governments will follow.
Chris:Yeah. I think the I think the European Union, loves kind of that being on the forefront of making rules like this. I mean, just think of GDPR. Nothing like it back back then when they when they came up with it. Same thing as this.
Chris:Look. I don't I think they're number 2 right now, and China has has kind of done this as well. But, you know, I still think they're on the forefront of this, and and I don't hate it. I think it's a good idea.
Ansh:No. I think I think for us as consumers, right, this this this is essential for us to be able to continue to function in society, and have faith trust really in in, you know, news media or or anything that's
Chris:Oh my god. How can companies future proof their data management strategies strategies to address, you know, evolving challenges in the data security and AI.
Ansh:Yeah. Absolutely. But we've been thinking a lot about this. Right? And the way we think of of data is is the foundational element on which any of these AI, related advances can be built.
Ansh:And so the questions, like, I like to answer these in terms of questions, right, which, help you assess the state of your data and the the readiness of that data to build an AI program on top of. Yeah? So first question is, is there a clear definition of key dimensions within your enterprise? Enterprise wide. Right?
Ansh:Dimensions such as customer. Right? Today, just for the data, forget about the AIMLPs, but just for the data. Are the rights to data and and access to that data, are they clear? Are those those, you know, are those data set governed in a way that you can be, you can you can audit yourself and feel confident?
Ansh:You know, is that is that data cleansed and standardized now sort of leveling up from what you receive from your systems? You and have you are you enhancing the data where you you have a higher probability of of extracting a signal from that data? Can you trace both dimension data and fact data to systems of origin? Like, do you have do you have command and control over that data? Because ultimately that, the, that that that that that, you know, when the question is asked about the, you know, biases or other things that have crept up into your AI algorithms.
Ansh:Right? Can you actually debug that for lack of a better word? Yeah. And then most importantly, are there established norms around the ethical use of data? Right?
Ansh:That to me is the, is the is the key question to ask because if you don't have established established practices and guardrails around the data and data models and how they serve your enterprise, then it's very unlikely that you're ready for, ethics and norms of use around AI models which are built on that data. Right? So I use these as as a rubric to really, you know, have a conversation about, are you ready with the fundamental model? And if you are, then great. You're gonna have a great experience building AI models.
Ansh:But if you're not, the idea is not to to apply breaks and say, you know, go build out a data governance program or or data, you know, a mature governance and privacy program that solves for all of these issues would really be aware of how much uplift or how much value you can get but get out of your AIML program because you also need to simultaneously work on your data foundations and move both of those balls forward.
Chris:I agree. I mean, it's some really great wisdoms there. And just some maybe parting thoughts. Are there any kind of emerging trends or technologies in data management and privacy that you believe, will significantly impact AI?
Ansh:Absolutely. A lot of it. A lot of it is gonna change. It's not me. Yeah.
Ansh:McKinsey, they quoted, a few months ago. I think in April, they released the the impact of generative AI, report, and it's I'm a big fan of that report. I think everybody should read it. They really look across industries, across use cases in those industries, and really identify these hot spots where they think, a lot of change is going to happen because of AI, but specifically because of generative AI. And, data management is one of the, one of the areas of change under that report.
Ansh:And what they say is 90% of how we do data management today is going to change. 90%.
Chris:That's a lot. 90%. That changes the whole
Ansh:work. Yeah. Over the next 5 to 10 years. Right? Because there there's there's still a lot of toil involved with data management.
Ansh:Right? If you if you think about just the just the the the variety and volume of data, it it grows every single day. And so keeping up with that requires a level of intelligence and and a level level of, well, right now, it it requires significant level of investment. So can down the gym so that that investment is kept that way even though, those those 3 v's or 9 v's of data continue to increase. Right?
Ansh:Yeah. But I'll leave you with this thought. It's going to be increasingly important as you build out these models, these AI machine learning, more and more sophisticated models. The the the fundamental question is going to be why are they making a particular decision. Right?
Ansh:Just like we question decisions that are made by humans, we need to be able to question decisions that are made by these algorithms, especially if they're high value decisions. Right? And so these decisions are driven by the data that we're feeding these models. And, therefore, as a data professional, it'll be very important for you to understand where did this data come from, what is its lineage in other words. You know, it may have been combined and and and sort of pushed through multiple transformations to get to its state, but still be able to trace it all the way back.
Ansh:Because if there is a bias in data, you've trapped in somewhere. And to be able to get to that bias and fix it is going to be akin to debugging software that we do today. Right? So root cause analysis and being able to draw these causal graphs of why something came to be in terms of a dataset, is very, very important. And that's going to contribute to model explainability where you will be able to then say confidently why the model made the decision that it made.
Ansh:And I think this is going to be it's it's already an area of research, but especially with large language models, you know, a lot more work needs to needs to go into this space. But to get the model right, to get explainability on the model, you need explainability in the data. And and that to me is a lot of what 2024 will be about.
Chris:Wow. That was really great, Anj. And, thank you so much for coming on to, another data driven podcast. Please rate and review us. So my name is Chris Detzel and
Ansh:I'm Ansh Kenworth.
Chris:Thanks, Ansh.
Creators and Guests
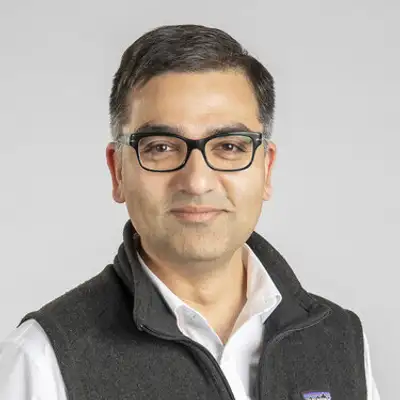