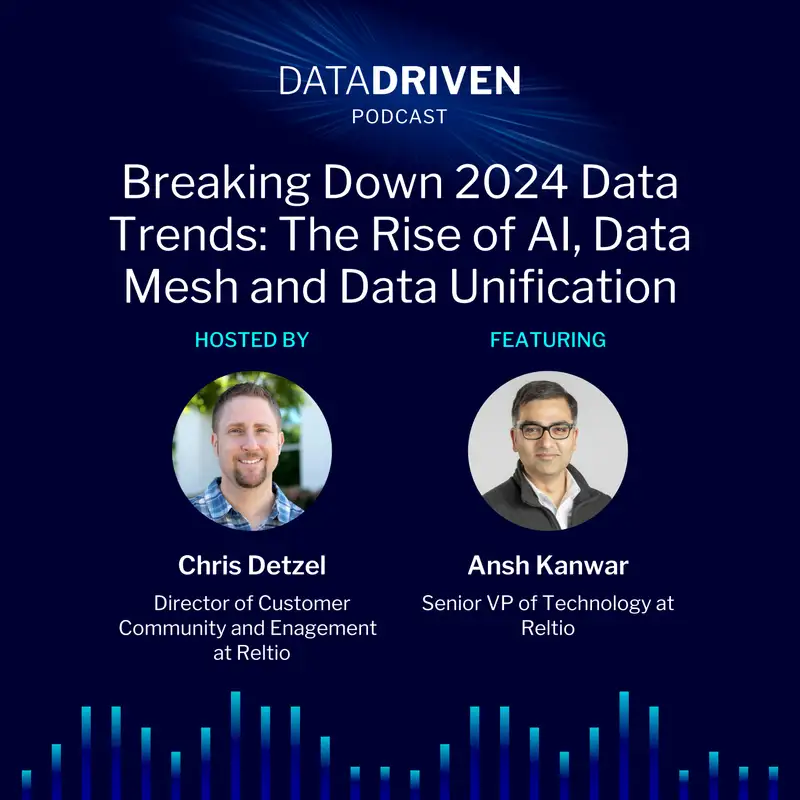
Breaking Down 2024 Data Trends: The Rise of AI, Data Mesh, and Data Unification
Welcome to the Data [00:01:00] Driven Podcast. I'm Chris Detzel, and I'm Ansh Kanwar. Ansh, how are you today? I am very well. How are you, Chris doing? Well, I'm really excited about our very first podcast. Um, and so what we want to talk about today is kind of this trends of 2024. Yeah, I got a new year coming up. Yeah, we do have a new year coming up and everybody wants to know what's going on in the data management field.
And so we have you on today to kind of talk about some of that stuff. What do you think? Yeah, yeah, I would love to would love to. This is this is. Yeah. All I think about every single day. So we'd love to share with you and, uh, with, uh, people listening. Great. Well, let's start with the first trend and it's going to be by no surprise at all.
Generative AI. 2023 was the breakout year. It's all generative AI. I think there's a rule that if you're at dinner with friends or heck, well, even with family, [00:02:00] Eventually generative AI will come up. It is just simply a matter of how long it takes to get to that conversation. I agree. And, you know, the question I have around that is, you know, how do you think AI or generative AI is going to change the landscape of data management, particularly in terms of automating tasks that were previously manual?
I think that's a huge opportunity there. Yeah. Yeah. Huge opportunity. My go to for this is a couple of, uh, reports that were generated by McKinsey, um, one earlier in April this year, and it's the economic potential of generative AI. Um, and they evaluated multiple different verticals and looked at the specific task items that are performed within that vertical that could be impacted by AI.
So, I will share a link. Do that, um, uh, with this, with this podcast, but, um, in there, there is a small piece around data management and they assess the [00:03:00] impact of just AI before generative AI, they said within the next 4 to 5 years. 70 percent of all the, all of the capabilities that, you know, that fall under the umbrella of data management, 70 percent of those would be impacted by AI one way or another.
With the new report on generative AI, they now raised it to 90 plus percent. So essentially the way we do data management today is going to be obsolete. superseded by. capabilities that are smarter, better, more efficient and really allow us to get our arms around this massive amount of data, right? The three V's of data, which grew into the nine V's of data.
Um, it really is a problem that needs exponential solutions. And I think generative AI really offers that. Yeah. So if you think about, you know, the traditional Extract, transform, load, right? Anywhere in the data universe that is, that is the constant, right? [00:04:00] So in terms of, um, that whole pipeline, the ingestion of data, uh, the recognition of what you're ingesting, uh, classification of that into and labeling of that data into sets that can then be treated intelligently.
For example, Um, a lot of effort goes into creating the right models, the right data models that can then be consumed downstream. Why does that need to happen in my hand? If you understand what the data is, if you understand what the business intent is of ingesting that data, the modeling should be a relatively straightforward and automated process.
Um, On the other hand, for test purposes, um, and, and not even thinking about AI models, but just for test purposes, there's a lot of synthetic data that is generated. And it's a hard problem because generating realistic data that is structurally equivalent to what you would actually get in a real [00:05:00] world scenario, that's a hard problem.
Um, generative AI is really good at this point in generating data sets that represent Reality more than something you would generate through through existing methods, right? So that's a that's another big area of change. But I think what is the most interesting to me is the broader implication of having these generative models.
Um, and the fact that they are amplifiers for. biases or flaws in the input data. And it's, it's really hard to, to reason through why they reached a particular conclusion unless you can, you can trace it back to, uh, certain pieces of data that caused that bias to appear in the first place. Right? So, uh, the broader implications in terms of having training data that is trusted, that is reliable, that then leads to models that are high fidelity, uh, whether you're training or you're, you're tuning, um, These models, you have to depend on your own data set [00:06:00] to give them context for your business, right?
And then taking the output of, let's say you, you have, you're using Lama or any other model that is just available out there. That inference has to act on a data set to produce value for your business, right? For example, if you're for marketing purposes, you're generating a list of customers, you're segmenting customers to then activate by sending them an email to encourage them to, you know, a particular sale, um, in, in, let's say the retail segment.
Well, you need a reliable set of data, trusted set of data. To which you can apply that inference, create that segmentation and, and push that activation out. That really doesn't change whether the model acting on this is a simple statistical model. It's a human being, or it's generative ai. So it's really this sort of, uh, uh, this overlap between this, the, the, the, you know, generative AI sort of speeding up all [00:07:00] of these capabilities and it's reliance on data, both for.
Uh, both for uh, bias free creation and training of these models as well as the activation of what comes out of it You've seen a lot of stuff and I really appreciate that Uh, and it's really good but and and you gave one kind of example and I and I really appreciate examples Can you provide a few more examples of how?
uh llms have improved data accessibility and efficiency with within organizations. Yeah, absolutely. I mean, and that impact is being felt today, right? That's not a future thing, right? That that we are at the we're climbing the crest of that impact right now. And where it really stems from is that 90 percent I think we've done surveys.
We've seen this from from other parties that 90 percent of even the structured data that is part of our, you know, most of our data landscapes is just locked away or it doesn't actually generate any value. Yeah, absolutely. And structured data itself is a very small [00:08:00] part of the overall data landscape, right?
Uh, you may know the exact numbers or at least the last service, Chris, but I remember being 87 percent of data is unstructured in any given company. Um, and The biggest benefit, I think, of generative AI is immediately to be able to parse and extract some value out of that data without having to put just a stupendous amount of work into making that happen, right?
A simple example of that is, um, To, uh, to create an email, it takes now, uh, an eloquent email, you know, explaining your case, it takes just a minimal amount of time, right? That's right. But now think about it on the other side, person receiving that email can also summarize it back down to what is the main central point of your email, right?
And so suddenly the amount of data, uh, is, is, you know, transmitting the wire is probably quite a bit because you generate it on the one end, but it becomes much [00:09:00] easier to. Uh, consume that large amount of data as well on the other end. So I think it's this sort of unique property where you can use, um, these models on both ends of a conversation and, you know, not even, we haven't even talked about multimodal models yet.
Right? So this is just pure text. So imagine the, the, um, the, the amount of efficiency that can be driven in, in, especially for, uh, information workers, um, just using code generation. Translation capabilities, first draft generation and summarization, just these four things, right? That's, um, that's, that's a very large amount of value creation that is happening right now.
Extremely helpful. And, you know, I feel like there's probably two or three podcasts that we can do on that one trend and we will do more. Uh, but I want to go to the next one and something I know you're very passionate about and we'll do another podcast specifically around each trend, but is this concept of data [00:10:00] mesh.
So in your experience, one, tell us a little bit about data mesh and tell us a little bit, you know, around how does the concept enhance data governance while still providing autonomy to individual teams? It really began as a reaction to how we were structuring these data pipelines, like everything in data goes back to data pipelines, right?
And so if you think about what, what is still happening for, for most enterprises out there is. data is generated in these transactional systems, whether they be your e commerce website or your billing or warehousing systems. And those then get shipped into one format, one form or another into your data warehouse or your lake house or, you know, whatever, whatever the analytical end of your, uh, data landscape.
Um, and then there is a consumption step, whether that consumption is through reports or it's, it's through an API to some other downstream systems and so on. [00:11:00] Right. But typically there are very different teams that are operating the operational systems that are creating data. versus the ETL teams that are getting data from the operational systems to the warehouse versus the people who are responsible for generating value reports, i.e. value from the warehousing system, right? And what happens is, along that path, you just lose context every step of the way. So, Zamak's insight is to think about both, not just the technical change that needs to happen to help with this flow of context, but really think about it as a socio technical problem,
i.e. what organizational structure would support a technology that then allows this context to go all the way from end to end. And what do I mean by context? Well, very simple, right? It may mean that, what is the definition of a customer for you? Right. Let in your, in your e commerce system is a customer, [00:12:00] somebody who's visiting your webpage is a customer, somebody who has a login or is a customer, somebody who's actually made a purchase in the past.
Right. So very simple thing, but unless the definition is the same. In your operational ETL warehouse and reporting context, you're not going to be able to produce a reliable report at the end, which allows you to close your business, um, sort of confidently, right? And so this leads to problems like. How many customers do we have?
Well, depends on how you define a customer, right? I remember, just quickly is, one of our CDOs, uh, you won't mind that I say this, is Joe DeSantos mentioned, that was the single hardest thing to do within an organization, is to come up with a definition of what a customer really is. And so I think you're, you know, you're exactly right.
So, keep going, sorry. And No, no, of course. Right? And, and, and it's, it's not just a definition of a customer, but then [00:13:00] also who owns the customer. How do you update that definition as you perhaps acquire business, you know, acquire businesses and or create new business units and so on and so forth. So it, it's, and the bigger you are as an enterprise, the bigger this problem is.
Right? Um, so back to the data mesh piece and, and the proposed solution there is really to think about the people who are generating the data as having the most context about that data. Right. And as an organization, decentralizing the, the, the, at least the technology, decentralizing that so that the organization closest to generating the data can produce the most authoritative information about that data.
So it really is about decentralizing the business into something that we know really well, which is data domains, different domains that may be a customer domain in the example we just use, or it may be a parts domain, or it may be, Supplier domain, right? And everybody will slice and dice these things differently.
Um, the [00:14:00] other pieces then to create these teams that are autonomous, right? And then try to create these teams that map to this, this, this data domain concept. And they really are the experts in that domain. And they publish both the data and also the metadata about that dataset, which then. Informs everybody else in the landscape on how to consume that data, how fresh is that data, how not to consume that data, right?
And then, um, over time, um, it, it, it becomes more of a, you know, less of a organizational problem and also more of a technical question, like, okay, but where do we publish this data and so on, right? So there is a concept of a self serve data platform that technically provides the data. Okay. underlying capabilities that are required for some of the concepts that we talked about.
But finally, I'm getting to the question you asked, which is how does it work? How does it help with governance? And I think the pillar there is, um, around, uh, you know, again, [00:15:00] conceptually, it's the same thing. If you understand what the data is intended for, how it was produced, um, you know, how frequently it's updated, what does latest copy of that data set and so on.
Applying governance to that becomes much easier because now you have the context that's carried with the data. You understand who owns that data. And if as a, let's say, a data steward or an auditor in a different part of the company, you have a question about it or you want some change made to a certain part of that data set, well, it's so much easier to go carry that out at this point.
Thanks, Ansh. And I know you're very passionate about that. And you and I actually have a podcast that will be coming out. Soon about data mesh and we'll go deeper into that data mesh concept and thinking, um, but the other trend and maybe it's more than just a trend is this thought around data unification, right?
So from your kind of thinking, what are the most significant challenges organizations face when attempting to unify [00:16:00] data from multiple disparate sources? So, you know, the question I ask around this is where does broken data come from, right? What are the sources of fragmented data that that really then there is a need to unify that source back?
So there's a very interesting, um, so Scott Taylor, the data whisperer, um, he's done a number of very interesting talks, but the latest one he did at Big Data London, uh, and we'll, we'll have a link to that here at the bottom. Um, uh, he, he take talks about an experiment where, uh, a company had their, uh, employees sort of input all variations of that.
They just said type in 7 11, right? And that was part of a bigger form. And they just wanted to see how much variation, you know, they could create in that single field, right? I was astounded. It was a slide full of eight point, uh, you know, sort of font, [00:17:00] just different renditions of what people put in as 7 Eleven when they heard 7 Eleven, right?
Um, and so, uh, you know, that's one example of where The intent is to, to, to get something entered one way, but it just, it just comes in, um, you know, 300 different variations of the team. Um, then you take the next step. You say, well, um, large corporations will, would have grown over many, many years through.
you know, perhaps through acquisition, um, the corporate structure changes over time. Um, and so data gets fragmented just along the lines of the organization. And, you know, we were just talking about the customer example, but think about suppliers, think, think about, you know, locations, if there's physical assets, all of that gets, gets, all of that gets fragmented.
But it gets fragmented for valid reasons, right? It's not like somebody wakes up in the morning and goes, okay, I gotta create fragmentation, the third I, I'm going to fragment my data [00:18:00] today. Right? , the third source, which is a big driver, is, um, uh, you know, the ification of our enterprises, or to coin a term, point, a phrase.
In other words, the more applications you have. that are, you know, that, that, that sort of rely on their own understanding of what the customer list is again, simple example, the more customer list you will have. And so, um, the, the, uh, uh, reasons for fragmentation, therefore, there are a lot, they're all valid business reasons.
And therefore, there is a need for an, for a technology or a group of technologies that are able to put that data back together to create this, Trusted uniform layer of data that can then be consumed reliably by other downstream applications without having to having to worry about all the fragmentation that happens, um, you know, upstream to which, um, you know, they, uh, they don't need to [00:19:00] have visibility or that's something that.
Uh, you know, at least downstream applications can, uh, take as a given that the data that they're consuming is actionable, trusted data. Yeah. So that's kind of the, the, the, the, the concept of data unification that we're starting to talk about as a broader umbrella. Um, and underneath of that, there's multiple technologies, right?
We, we know these technologies by multiple names. We, you know, the simplest version of that is entity resolution. Anti resolution being, are two things, or two addresses, two first names, two profiles of customers, are they the same or not? And this really, um, you know, it, it sounds, uh, like a very simple concept, but a very powerful, um, rendition of this.
I was just reading this morning, uh, an article in Forbes about, um, Sam Altman's next big idea is to create this universal ID. It's, [00:20:00] uh, it's based on India's Aadhaar card system, which really is, is, you know, the equivalent of, you know, think of it as a social security number on steroids. And every citizen of India has that number and they need that number for any transaction, right?
And they need that identity card, which has biometric scanning for, for any transaction, public transaction. Whether that be financial or not. And so, if you have a solution like that, if all of us had those numbers and it was socially acceptable to use that number to prove your identity, well, there would be no data fragmentation, at least for that space, right?
It'd be really easy. Like, what is your number? Oh, great. You know, prove you are you by biometric identification and we're good to go. But in the real world, you know, across the world, So, with that Isn't the, the, the reality, right? So we need these systems that are really thinking about tying these fragments of information about identities, about different, different nouns, if you will, right?
A [00:21:00] lot of suppliers and so on. And, and, and it, it, it, it is essential to how our businesses function. And one other question that was really good. I love that, uh, universal ID. Example. Um, can you share like an example of a success story where, you know, data unification had a tangible impact on an organization's business outcomes?
Yeah, there's so many examples that come to mind. Um, but we'll talk today about, um, one of our customers, uh, an athletic apparel retailer. Um, and they. Uh, they, they, they decided early on to put Reltio in as, uh, is that sort of trusted layer which has an authoritative, uh, which is the authoritative system for customer information.
And over time, what that has allowed them to do is to build business processes, all these operational processes on top of a confidence, a very high [00:22:00] confidence that has allowed them to do. is to build applications on top of this very solid layer of information that is evergreen and has the latest and greatest information about their customers.
So what do we mean by that? For example, if you walk into a physical store, you may have, um, uh, you may get help from an attendant who has an iPad and they ask you some basics about you, maybe your last name, maybe your email, and they're able to pull up your entire profile, your, your purchase history. They may be right there and then able to offer you a discount for a particular, you know, a personalized discount, if you will, because you bought a certain kind of apparel in the past, they could discount, uh, you know, a different color or a related piece of clothing, right?
Now, if you want to return. What you bought in the store online while their warehousing system has the same exact understanding of you as a customer, and therefore there [00:23:00] is, uh, there is no gap between the moment you bought something in the store and when they're ready to accept returns, right? Um, if you, uh, interestingly, this company acquired, um, uh, an unrelated business, which was in the exercise space, exercise equipment space.
And so. As they ingested the business, they now have a much bigger set of customers if you combine the, you know, the existing customers of both businesses together. And they have an extensive program to then go cross sell either apparel into the exercise space or vice versa, and they had exercise equipment in their physical stores.
So all of this intermeshing is possible if you have this good understanding of their customer base right there, especially for those customers who happen to be existing customers of the two businesses before the merge. Well, they got a very seamless experience because they knew exactly who those were and they treated them very, [00:24:00] very, um, uh, different from a customer who was only on one side of the equation.
That was really good. It's funny that you bring that up is, you know, the expected outcomes from a customer is that, you know, whenever you go to a store you buy something, but then you want to return it, but you return it online. Even though there's two different systems, people don't understand that. The customers, I remember my wife being like, I don't care.
Fix it. Figure it out. Like, I mean, you should know who I am. You know, that's just the expected outcome, uh, from a customer. Right. And so, um, but it's not easy. I tell her all the time. I was like, you know, it's not as easy as you, I don't, and she's like, I don't care, you know? You know, in marketing circles, we talk about personalization to an audience of one, right?
And a lot of our customers are moving in that direction because they have the confidence that with a data layer that is consolidated and really, [00:25:00] truly is that one view of their customer, they can build multiple operational processes seamlessly together, right? Yeah. Um, there's so many examples I could give, but, you know, Arch, That's really all we have time for today is those three 2024 top trends.
There's a few more that you know, maybe we'll get to at some point soon But it's been very good and everyone. Thank you for coming and tuning in to another data driven podcast My name is chris detzel and i'm on can work Thanks
Creators and Guests
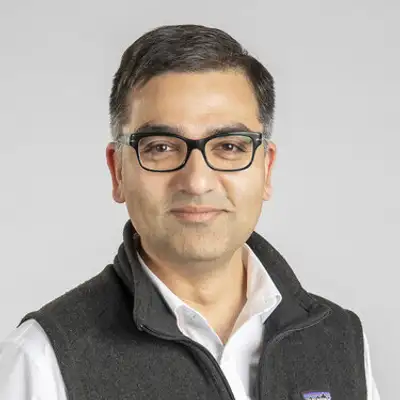