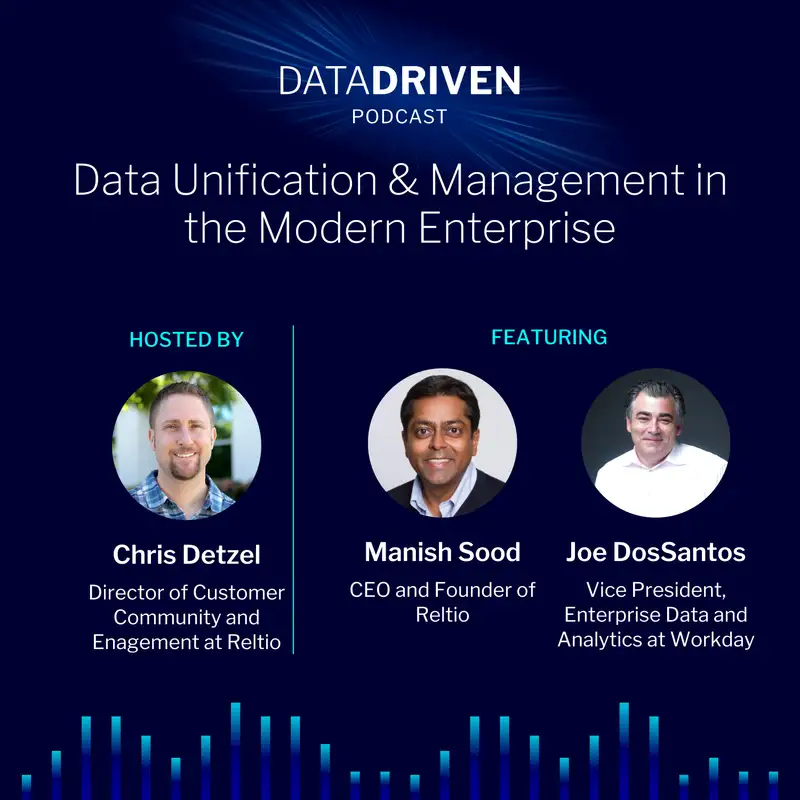
Data Unification & Management in the Modern Enterprise
Welcome to another data driven podcast. My name is Chris Detzel and we have two special guests that I'm really excited to to get to today. And so our first guest is Manish Sood. Manisha is the CEO and founder of Reltio. How's it going Manish? Great, Chris. Great to be on the show.
Thank you for having me. Thanks for coming and we have Joe DeSantos, he's a VP of enterprise data and analytics at Workday. How you doing, Joe? Horrific. Good to be with you, Chris. Hi, Manish. Yeah. Hey, Joe. And so today's topic is we'll go deep into kind of the keys to a modern data strategy and what all that means to to Joe.
So what I'm going to do is hand the reins over to Manish and let him kick this off. Manish. Thanks, Chris. Joe. I've known you for a long time, and I've been [00:01:00] I look at your career in data, and it's truly inspiring. I just wanted to understand what attracted you to data.
You know why the current role that you have at work. What's been your journey in this process? So I spent the first 10 years of my career doing ERP implementations, which was putting data into systems that you certainly found out later on were very difficult to get out of those systems. So it was it was about, it was an age of process improvement and about, making sure that you could get past the cost of the system.
And then, in the late nineties, people started to say, there's really some. some goodness here in terms of trying to figure out how to make this work. I built my first data warehouse in 1998 and I was with you at at Cyperion for a few years cutting my teeth in the MDM space. And then I went on then waves come, big data waves come.
And so I started up Big data practice at EMC, and [00:02:00] it's really what they hear that you start to, to develop these fascinations with these use cases. We were, we helped an electric company figure out where all the electrons were going in the grid to detect theft in the grid. We helped to determine for for banks in Brazil where the.
ATMs were about to be stolen, these really cool things. You started to realize that this was well beyond the dashboard and just some really kind of life changing types of things that affected real life. So then I got what I think is a really life changing opportunity. I was at Petiti Bank and I was able to take a job on the other side of the desk, really move from consulting to really owning a data infrastructure for 15 lines of business.
And I learned a lot about what it really takes. And I started to think about the my axiom that the job is 10 percent technology and 90 percent psychology. It's really about trying to get people motivated around a common objective. And I really learned a lot around what not to do sometimes too, in terms of if you build it, they will come things.
That was up in Toronto and I was living in Boston, which was not a [00:03:00] great place to raise children in that manner. Or so my wife tells me. And so I I had a good friend. Paul Barth, who encouraged me to come to Click, where I was able to take a lot of the lessons learned on the technology side, but really start to create a business view.
I became the first chief data officer, chief data and analytics officer at Click. He only started to do some things around trying to make sure that we could focus on business outcomes, focus on a data strategy that was really a business strategy with data. And that was great. I was there for five years.
And recently about six months ago, I came to work day. It was an opportunity, just a great company 10 times the size of click. So a really amazing opportunity a classic situation to bring. Lots of different business lines with lots of different ways to analyze data, real hunger for data at Workday.
And this is an opportunity for us to really streamline the way we provide access to that data and kind of launch ourselves into the new AI era. That's great, Joe. Throughout [00:04:00] your career, you've seen the evolution of how people have been thinking about data in order to serve business.
And today, more than ever before the talk about data is, I would say, at an all time high. in Your opinion, what do you attribute it to, and also how would you ask people to think about the modern data strategy that they should be putting into place? It's it's funny. There are these things that come along that I feel are the lifeblood of data people.
These statements like data is the new oil or the data scientist is the sexiest job of the 21st century. And it starts to in some places create the wrong expectations, right? So Oh, if data is the new oil, but basically I just need to stockpile oil because it has a price to itself. So I'll stop.
Yeah. And so you, you get these the metaphors break. They also start to realize they help the, they, the [00:05:00] business does not necessarily care about data mechanics. They care about outcomes and they're pretty interested in terms of your ability to do something. But really it's like you own a steam shovel, right?
What do you want to, what do you want to build? Because a steam shovel in your driveway doesn't particularly help. So data and analytics are all tools. And I think that's been a long journey because over the past, 10 years we've come out of Lake architecture, which is really about if I could stockpile data, I could be like Google turns out that really doesn't work.
And so here, I think I've turned the page on kind of what I think are key principles for a modern architecture and a modern strategy. And, I Think the first might be obvious, but it's about being value based. Bill Schmarzo has this great saying that he says companies don't need a data strategy.
They need a business strategy that that uses data at its core. And I think that's really important. How do we help executives connect the dots to the way that companies make money, to the way that the strategy is meant to be employed? So at Workday, for instance, we have an international growth strategy.
We have a product strategy. We have a [00:06:00] mid market strategy. We have a new emerging product strategy. So all these different things are things that I talk about every day in terms of what would it mean for us to be able to penetrate these markets differently and what kind of data and analytics would be required to do that.
It has nothing to do with analytics. It has to do with this vision. Our chief marketing officer says, you know what I would love to be able to do. I'd love to be able to know what would happen if I diverted 20 million of spend from us field marketing for one product into Japan brand advertisements. What would happen to revenue and when that's the kind of thinking that we need.
It's really around what is the outcome and who are our champions that are going to sponsor that outcome. The second thing I think is about having a real clarity around. methodology and really moving to an engagement model that is iterative and agile. It's so easy for us to talk about failing fast and being glib about that stuff, but this is a developer mindset.
And how do you drag your business through that? So we all have these experiences where, you know, you, the business says, I want a report [00:07:00] on fill in the blank and you spend three months building it and you come back. And they said, that's not at all what I wanted. And if all you needed to do was come back every two weeks and say, how about this?
No, tweak that. How about this? Tweak that. How about this? Tweak that. So we're we're working with a methodology that is anchored on round a principle that Gartner calls fusion teams, which is really involving the business in this really iterative process where every two weeks you show them something, build and iterate.
And it is actually Not that foreign a concept for our development community, but actually fairly remarkable for the business. If you think about a product management strategy for a company, sure, they might be agile, but you don't care. You just want to talk to the product manager about the roadmap, right?
So we're really bringing people into the sausage making. I think that's a big deal. Number three is I think that we need to really be focused around this idea of data availability. I think there's a lot of talk in the marketplace around things like data meshes and data as a product and these different concepts, what they have in common is that.
As we start to move into the [00:08:00] 21st century more deeply the winners and losers are not going to be dictated by whose data scientist is better because AI is pretty profoundly better than a human being. So how do you make it so that the data is just available and of high quality and there on the shelf?
And how do you create the apparatus for creating reliability in that data and also the distribution method for that data? Fourth, I think you clearly need to have some chops in analytics. We need to move well beyond dashboards into, analytics at the edge. I think we all want to have the Amazon experience.
People who want that also bought that the Netflix experience. Since you watch that, you should watch that. And most of us don't get that in our professional lives. We should. We should give that to our customers. We should give that to our employees, but there's some thought process that's required to really understand the processes that are driving and some laser sharp focus on that.
Getting analytics that are delivered at the edge in the process or you can catch somebody in the act of deciding, right? And then that, that last [00:09:00] part is really number five is making sure that there is this focus on action and the measurements of that action. This is great stories about.
Back when the internet was new, back when Facebook was new they had FarmVille and they used to change the color of the sky in some places, right? And they'd measure the impact, right? I always feel people don't go back and measure whether or not the thing that they did was actually working.
And you don't measure analytics, you measure outcomes. So the analytics drive the decision, that decision drives an outcome and that, that outcome should be measurable and you should be able to tweak. And if you don't know what you're measuring, You will start to lose interest to your business stakeholders, because what you do is already a weird science magic thing for them anyway.
So that's it. Value based orientation, methodology, data availability uh, analytics capability that go to the edge and the ability of understanding those actions and measuring the impact of those actions. So Joe in order to drive to business value, it seems that Making sure data is available easily accessible, [00:10:00] becomes a key part of it.
So is data products the way to think about it? And what is the difference between data products and the traditional approaches that have been looked at in the past? Yeah, this is, I think this is one of the best evolutions of data in a long time. And it's so sensible, but so elusive. If you think about the traditional way by which we do complex analytics, there's a Batman and Robin of a data scientist and a data engineer.
And off they go and they create their own versions and they impute data and they make things up and then they great make these rate models. And there's no reusability to that because the data model that was just created was specifically created for that very use case and nothing else. So how can we do, how can we put a process together where we replace that that kind of quick sloppy making of data for a purpose to something that is durable and reusable.
So I think that. We need to think about use cases that that are real, that we might start with that, but then they get hardened. And this data becomes [00:11:00] something that has an SLA, something that has clear expectations around the quality standards, about the reliability, around the delivery frequency.
It should be self describing. aNd then the other part that I think is really important around how we think about this projects have a start and an end date. They have a budget. Products don't right. Products basically, if you declare a product that's big enough, like a customer insight or a go to market or enterprise metrics, these things have wide places to go and you need to manage them.
Just like you manage a consumer product, it's got a backlog. You'll never be able to get to everything all at once. So you have to have product management principles around having a person who sits there and the life of a product manager is tough. Everyone's everything now and the customers want better features and the new prospects want different things and data should be no different.
So you need to manage the politics of that. And have it be permanent or at least semi permanent, right? So I think those are the essential parts of what a product is to create this to create this data that [00:12:00] never stops. It's a tap that you turn on and the data keeps coming. I think where people are headed, which really turns this from a concept into something that really becomes real.
Traditionally people think about data products as something that you pay for. I'm not necessarily sure that's has to be true, but for sure, you want to monetize data, the outside world if possible. And I'm starting to see some some movement internally, shouldn't we cross charge a business organization for this one?
It's not free to make that data product. So how do I re how do I reclaim the cost of the creation of that product? And then the other part I think that is emerging is. It's nice to have products, but what does it mean to make them available? You're starting to see these, the marketplace emerge with solutions for a marketplace, a data marketplace.
So some of the cloud providers have made it so that you could buy, third party data sets, but I think even internally, wouldn't it be great if you could figure out. That somebody's already solved this problem. Somebody's already curated this data set and you could reuse all the assets that are available in your company [00:13:00] in a very kind of social way.
So imagine you go to, this is what we're building at Workday, right? A very social fabric that is about connecting people with each other so that they can never build something that's already been built before. And what would be some examples that you're thinking about or have seen in the past?
That everybody should think about or focus on as a starting point. So we talked a little bit about how they need to be, the right size, it's got to be big enough so that it isn't, it isn't boring to people. It's got to be small enough that it isn't too lofty, right?
So I think something that has, almost everybody has, it has something that they want to know about their customer's journey. How do they buy? How do they adopt? How do they renew? How do they come back for more? And there's so much in that journey that it makes for a really interesting product.
And we had a product like that at Click. We're building one like that at at Workday. And the idea here is that there's lots of different dimensions and lots of different places to go. [00:14:00] And you start off with some really mundane things like what is a customer? How do I count my customers? Do I count hierarchies or ultimates?
So I think that was something that was very successful because it created clarity of nomenclature, reliability of data, and a lot of really interesting things and insights about how customers come to you, how they purchase from you and how they adopt your product. There are also some things that are, I feel like there are a lot of financial metrics that are.
A highly reusable. So in the software space, one of the most important metrics you could have is annual recurring revenue. He has a subscription business. You're trying to figure out how to analyze that business. And so one of the things that happens is that the actual number itself isn't often that difficult to calculate, but the actual analysis under the covers is really quite challenging.
And at at Workday, we encountered a scenario, for instance, where let's say there's a customer who is set to renew with two weeks to the end of the quarter, and they're in the middle of negotiations. And so you say, it's fine. We'll give you a couple weeks [00:15:00] leeway, and then they renew with the next quarter.
Did that just become a 0 contract? Was that a churn? Was that a decline? Was that a pen? So you have these definitions that people never even conceptualized, right? And so how in the world are you going to make a good churn model if you haven't even defined what the heck churn is in the first place, right?
So we have really dug in on that one at at Workday. And really done a lot of work to standardize how we think about our revenue. I also think employee is a great product, right? So if you think about employee data in terms of how you attract talent, what kind of people stay, what kind of people perform and developing models around your data.
You can also provide insights that give back to the kinds of training for your employee data too. So customers, financial metrics, employee data, those are all really, straightforward ways to start as long as you build use cases on top of them. So what I just said was basically, the meat of what the product is, but I just finished saying it's worthless unless you can really paint imagination around use cases that senior people are going to want to do with [00:16:00] that data.
If they can't do today, One of the key elements that you are talking about is the reusability of data. And especially when you think about these core data domains, whether it is customer or product or supplier type of information multiple use cases touch this type of data, but at the same time, in the past, people have, gone down the path of, oh, for this use case, I'm just going to go build everything that's needed to service that use case, including the set of data that I would capture and use for it.
And, you've been in the financial services industry, I'm always fascinated by the number of use cases that touch the client data. Yeah, just think about compliance as a scenario. KYC, AML, compliance related things for onboarding that need to happen. Customer experience has a separate adjunct to it.
In all of these cases, at the heart of it, it is the same client data that has to be reused. But [00:17:00] everybody went down the path of use case specific information that they needed to leverage. First of all, as you think about reusability, how are organizations going to make that shift, where they come to a conclusion or go down this new path where they're promoting the reusability of the information?
Is that something that companies are starting to move to or think about or. How are you thinking about that reusability aspect? It's a really fascinating question. So I spent the first 10 years of my life in manufacturing. And one of the things that is clear in manufacturing parts is when you make a product, you have raw materials, you create semi finished goods, you store those semi finished goods and you use them to accelerate the products of your finished good.
And the way that you manage your supply chain, pennies matter. Cost mattered. And so you find that this paradigm is very easy for manufacturing companies to understand. Oh, you're talking about some semi finished goods. [00:18:00] Yeah. Yeah. Yeah. I'm talking about reusable semi finished goods that you can put on the shelf.
And then you get to financial services. And there's no actual physical product. So people can't see and touch all of the waste in the supply chain. There's no history of supply and chain banking software, same thing. And so I do a lot of storytelling around, how those, how we can get to the same value with different cost takeout.
I tell the story all the time around. There was a case I read about in business school many years ago that was about Benetton and how they used to not necessarily know what this year's hot color was, and so they'd put their. Their clothes all in beige out in the warehouses and paint. They knew what the styles were going to be, but not necessarily what the colors were going to be.
And so it's a kitschy little story. I don't get where you're, Oh, cause you can get some of the stuff done and then I can make it really fast at the end. Yeah. So I think that. An organization that's looking for cost takeout every time the economy is bad is a good time to talk about reusability, right?
Same [00:19:00] value, less cost. Isn't that what we're talking about? So I think that is part one of the story where everybody wants to have efficiency in their analytics as opposed to, we're getting through this decade where everyone's got these really cool algorithms that they're really having a hard time monetizing.
But I think the thing that's really going to change everything, is a I think that the idea is that you can deploy a I really easily against data. You trust and you can't use it at all against data that you don't. And this is the dirty little secret. Like one of the things that happened with chat GPT is when it was released into the wild and the training of the humans became not just some people in the bay area but actually, the broader population, it suddenly couldn't answer questions that it could before, because the feedback and training it was getting wasn't as good.
And I think that this idea of, if you want to have an AI strategy, you need to have a data availability strategy. Otherwise you're going to waste all your time and energy. Yeah, step one of that question is, yeah, in a [00:20:00] world where 80 percent of your time and analytics is spent getting the data, don't do that.
And you can be a hundred times more productive. And you can deliver data for cheaper. If that's attractive, great. If not you need it for AI. Otherwise you're just going to spend all your time in silos, frustrated and not get any value out of the AI. And how much in your opinion is this?
Anchored to the technology itself around data versus the methodology that you need to adopt and work with. What's the mix there? Yeah, it's interesting because I think that first of all, on the technology side, there's, it's I'm going to reveal a big secret here, Manish.
The cloud is this big thing. We're really going to, it's going to be big someday, right? I think that if you're not trying to figure out how to move all these things into a cloud as a service oriented solution, then you need to start, you need to finish, right? So I think, but apart from that, you can see that there are lots of different patterns.
There's federation technology, there's cloud data warehouses, there's lakes, there's different [00:21:00] ways that you can store and access the data. So I think that the technology matters a lot less. Then the methodology, how are you, how do you build in a procedure for reusability every time you do an analytic, right?
So for us, if you're trying to build, there's always somebody that has to go first. And the question is how do you make it so that they're not. They're the last person to go first. So let's say that there's an analytic. Let's I'll take my error example. I'm trying to do some financial analysis around churn.
And I spend six months doing this analysis and building this data model to support my analytic. Super. The next step in our methodology really has to be around. Hardening that, solidifying that, wrapping an SLA around that, making that code performant, that's a muscle that we need to build, right?
Productionalization and operationalization isn't just about getting the analytic right. It's about getting the data right and permanent, and then putting it on a shelf so everyone finds it. And this is going to require a whole kind of Turning the DevOps [00:22:00] principles into DataOps principles. How do I make it so that the code is QA tested?
How do I make it so that it's on an SLA and monitored? How do I make sure that it shows up in a catalog as available? That's a combination of governance skills and DevOps skills and By the way, oftentimes the data scientists don't even know those guys, right? So think about that language gap that exists between data governance people and data science and DevOps people, right?
There are three different language skills. One's kind of the language of what data means, ontologies and taxonomies. One's the language Of, technical infrastructure and how do you make sure that you can put things in Kubernetes and how do you deploy things in an architecture that works a certain way?
And one is the language of the algorithms themselves and the ML ops, right? They need to be brought together in a common methodology so that they can start to make sure that whatever they do becomes reusable to the person that comes next. So that's my mission is making sure when my wife asks what I do for a living, she says.[00:23:00]
He's a professional translator. He helps technologists speak English. So we're helping not only the business people understand what's happening in the analytics world, but actually these three different legs of the stool really need to work together. And that's a learning curve. That's a learning curve.
tHat is definitely a big learning curve. And, if you look at the last 10 years it almost seems all of the data science investments have been completely separate and distinct and on an island by itself. So bringing those things together in the service of business is extremely important.
But let's switch gears to the exciting topic of AI. You mentioned AI in service of business and delivering business value putting it to use there. How about putting it to use for data strategy? Where do you see its role in the data strategy, the things that you're trying to solve for data so that you could, have these data products ready available?
For use, do you see a role there for? [00:24:00] Yeah, it's a really good question. So let's take a step back and think about some of the examples when we close our eyes and think about who's really good at analytics. There are people who are laser sharp focused around a particular. Moment, right? So Amazon focuses on the moment that you buy something and what you're going to do next.
The notorious target story is about knowing when you're going to have a baby and changing your body your buying behaviors. Netflix, when you buy a movie, this kind of laser sharp focus really helps. It will help again in the next cycle, right? What is it that we're going to do with AI? If you throw in a whole bunch of data and let data loose your business will not proffer because you can figure out the difference between a dog and a wolf, or you can figure out which of the stop signs are on the, on your grid here, right?
So it really needs to be focused at outcomes. One of the things that is fascinating about Workday's strategy. Is that because the company has been so laser sharp focused on the job of the HR professional and the financial professional, they've been able to start to think [00:25:00] about use cases that are really relevant for that particular role in stride in terms of how they do their work as a process.
So I'm a person who's about to hire somebody. What if I could automate the process? Of writing a rec with generative AI. What if I could actually automate the process and accelerate the process of writing a job description with with AI? What if I could actually collect feedback about somebody and and accelerate the process of writing reviews or doing calibrations?
Like all of these things are very targeted and very specific to a specific moment. And I think that's, what's going to, that's the first step of being successful in companies is making sure that you're laser sharp focused on a moment that you're trying to change. Then it's about, how do you make sure that you have the right understanding of how that process will happen?
What screen will they be looking at? You'll notice I haven't said AI yet. We haven't even started talking about the actual method by which we're going to do this. So I think that getting the business to be clear about what they're going to do then it starts to move into the data availability because actually with generative AI, [00:26:00] that part's getting pretty easy now.
So if you have a specific moment in mind that you want to change, if you have an application in which that transaction is going to live and you have the data that's required to support it, deploying the gen AI on top of that should become straightforward, but that's the path, right? Getting business to focus.
By the way, this is one of the things that has been both easy and hard for financial institutions, for instance. So I think that most financial institutions are really good at risk management because of desire and because of regulation. And so you get really sharply focused on these different use cases.
And then beyond that, there are a thousand things that you could do. And this kind of all these things that you could do, what do you do when you talk to somebody, when they walk into branch, what do you do when you talk to somebody, when they hit touch an ATM, online banking, mobile banking, there's so many different scenarios, there's personal banking.
And so what happens is that they, This lack of focus means that you get this kind of heroics of data science that we've been talking about with a lack of reusability, right? So the secret, I think, to good analytics and ultimately good AI is around alignment and stick [00:27:00] to itiveness uh, and doing one thing a hundred percent as opposed to doing ten things ten percent.
But with the, with so many possibilities, with so many things that can now be done easier, faster, maybe not so much as cheaper, But when you think about that and this notion of focus that you just talked about, how do you look at the future? What are the trends that you foresee in data and product and strategy around those areas?
I am excited about the emergence of the data marketplace, so I think that one of the things, if you extend, we've been talking today about the metaphor of thinking like a supply chain data, like a supply chain, right? So data supply chain is fundamentally about getting data to the shelf.
How do you get it off the shelves? How do you get anything off the shelf these days? You go to some app and you search for it and you look for metadata that's germane to you and you find it. And you interact and you look at how many [00:28:00] stars have been put on there and you look for good reviews and bad reviews and data will come this way.
Data and analytics will come this way in terms of our ability to consume. I think that fundamentally the role of the chief data officer, we, there's Tom Davenport and Leandro Delamule wrote a piece in the Harvard Business Review last year around the shift from offensive, from defensive to offensive strategies and the mix thereof.
So I think that financial services spurred the, chief data officer role in these highly regulated protect me kinds of places. But at the end of the day, that moved to, we're going to make some money. And I think now as data and analytics become more commonplace, it starts to shift again into this kind of brokering method.
So what's going to help people broker data? Marketplaces. So I don't have a supply chain that puts it on the shelf. And how do I have a marketplace that helps people take it off the shelf? So I think that's going to be a big thing that's going to affect how we interact with data. no, one, one question in that particular area that comes to mind, this notion of a marketplace has always been something that people have thought about as an external [00:29:00] facing concept.
Versus, a lot of what you're talking about is even within your organization, making data available in this manner so that you can have commerce associated with it. A little bit of what you touched upon was the cross charge type of concept. So how do you bring that into the organization as a way of doing business versus, every time somebody talks about marketplace, it's always about.
A party that sits outside your four walls and how you transact with them. Can you talk about that a little bit while you're on the topic of marketplaces? Yes, so we have started to talk about this at Workday in the context of creating a community, right? So at the end of the day, if you want to know something if somebody has a question about how to do something, they put it on Slack.
Then it's lost forever as soon as it's gone. What if that interaction was about... A knowledge base. What if you could find somebody that does really cool stuff and you could follow them? What if you could actually access the data and search for it, right? [00:30:00] So it's about community building.
So a lot of what we're focused on now is change management in terms of if I'm really a broker. It's not me in my service to you. It's you helping service each other. That's the concept of data mesh, right? It's not about somebody in the center arbitrating everything. It's about creating a fabric for people to communicate ideas and algorithms and data with each other, right?
So some of that will have to be done in the physical world, like We're going to have a data science day once every year and people will come together and show off what they did. There'll be community calls and then there'll be this kind of social fabric that we build and embedded in that is all of the metadata that we've been carefully storing about this data so that we can actually track and use it.
But yeah, this is absolutely for internal and external use. And by the way, not only are we, we are not right now in the business of monetizing data to the outside world. tHat's something that we might, and we might do later on, but for now, it's mostly the other way around. How do we capitalize on the outside world data to make sense of our company and our business, right?
So I think that's a, it's a psychological move. We're trying to build community and bring people together and the marketplace is [00:31:00] one of the legs of that stool. Got it. And, going back to the predictions so you were onto your second area that you thought was going to be relevant.
Yeah. So I think I think that we talked a little bit about the composition of a product team. And I think what's going to happen is you're going to have growing product teams and shrinking data science teams. Maybe data scientists wasn't the sexiest job of the 21st century after all if if chat GPT can do the job of that person.
But if you start to think about the ratio of time spent, we need to spend more time getting the data, so that we can, Okay. Capitalize in the less time being done by an AI uh, robot instead of our data science team. I think that data product owner is become, is going to become a much more common job title.
I literally just tried to hire a job a product owner. I did hire one here at Workday. And just in terms of comparison, I had 150 applicants in the first five days for a data governance role. And I had four for product owner and none of them had any data experience. So this is new, right? We're talking about it as if it's [00:32:00] commonplace, but it's not.
anD then one of our technology thing that I think is really going to be interesting is we are talking about AI within the concept of how it's going to help us with insights and analytics. I actually think it's going to help us with the actual construct of the products themselves too, right? So today somebody somewhere is making a metric.
Somebody somewhere is making a data model. In theory, AI should be able to unwind what's been done, figure out, dissect and make a proposal to somebody about how you're supposed to wire that data together so that everything gets easier, right? So that's, I think this is just starting to happen.
I've started to talk to a few startups who have been talking about AI and the concepts of transformations as opposed to analytics proper. So as companies think about this transition, how would you suggest they prepare for this? So if you think about I mentioned this idea of getting people to talk together that don't speak the same language, your technical people, your data people, your app dev, your UI design is [00:33:00] going to become a big deal, business speak.
I think that the thing that you'll need to do as a data leader is make sure that you're rounded out. We all come from one place. We all come from either a governance background or analytics background or something like that. It's really important to round out your skills in business acumen and dev and UI take training.
On on design thinking, different kinds of things like that. That's really important. Number two, as you start to think about lining up that use case. Pick somebody just as much as something. So my running joke is I'm like Bette Midler. I'm just the wind beneath your wings. You need to have a champion that can really crow about the use case and its impact to your business.
We're in the, we're in the business of hero making. And we want to make sure that we are building something that is of matter that gets really real marketing buzz inside of the organization that solves a business need and gets your momentum going. And I think that the other thing is, as we start to think about this product [00:34:00] mindset, just thinking about how one interacts with products.
Physical products and digital products in your life. How do you buy something online? Physical digital? How did you buy that app when you downloaded it? How did you buy that pair of pants when you last wanted it was in the store? Was it on an app? How did you return it? All these different things, because ultimately.
Most of the metaphor of supply chain has already been created. And it's just a question of trying to make sure how you use these metaphors to explain it to your business and how you actually employ them and put roles in place that are like, why would you need somebody like that? Because we need to handle returns.
What do you mean we need to handle returns? Oh, I'm sorry. I'm talking about a quality issue. So somebody actually diagnosed a quality issue and they returned it and they want somebody to fix it and they want a new one, right? It's the same metaphor. And so I think, I think that's how I'm thinking about it.
So getting rounding out skills, making sure that you're picking the right use cases, and then really doing some thinking around this is all evolving. And I look forward to reading and writing things around what other, what are the [00:35:00] roles we haven't been thinking of that are going to be required in this framework.
So Joe, there is so much to so much more to talk about, but one last question for you as we wrap this up is what advice would you give to. Professionals out there who are thinking about their career and thinking about, how they should be involved with data, especially with this context of data products and data strategy in mind.
And, on top of that are there any specific skills or knowledge areas that they should focus on as they think about developing their career? Yeah, I'm going to say something. Maybe. I'm not sure if it's stupid or profound. You can tell me many. I think that people think, Oh, you really need to start understanding how large language models work.
I do not understand how my mobile phone works. I do not understand mobile signals. I just use it. This is a moment for harnessing the work of other people. So how do you start to harness What's being done in Silicon Valley and other places for your use. I would say there's four things.
[00:36:00] Number one is really stay close to the business and develop a deep sense of acumen. I had our head of FPNA said, I want to close the books in two days, make it happen. Okay. Now we have something that anchors our vision. We have a head of marketing who has a very, a clear picture about marketing, diverting resources a certain way.
I need to be able to have a language, a conversation with them and know what they mean, because if I was like natural language, they wouldn't know what I was talking about. So business acumen, it's a really big deal. Number two is making sure that you're pushing more and more into cloud technology and understanding what's available out there.
Number three. Increasingly, the old is becoming new with respect to data governance. It used to be like, command and control and all sorts of things. But if you think about what it means to have a supply chain, I need to be able to have discovery and tagging the creation of a semantic layer. So if you're not well versed in terms of how data governance works, it's going to be really hard to put the barcode on the product on [00:37:00] the shelf.
Because that's the thing that has all the data that you need. And then the last thing that I would say is It's no longer acceptable to just be a person who knows about data in a post pandemic digitization world. You need to understand about apps and digitization and how people consume them, which means you need to understand design thinking and how people are going to actually interact with the analytics to make decisions differently, whether that's an individual person making a discrete decision or a company making a broad based decision.
So I would say, focus on the things that are about the adoption. Of the AI, as opposed to how AI happens. Of course, you need to be well versed so you can have a communication with your own team, not sound like a fool, but really your job is to make sure that can breathe life into a real life business scenario.
Joe that thing that you talked about where you're not going to try and understand how to create the cell phone, but be an [00:38:00] effective user of it. I call it the layer cape of innovation where. There's, if you think about all the capabilities that are available today, how do you put it to maximum use for the maximum velocity that you can bring to the table?
Because otherwise everything is moving at such a fast pace that you're going to be left behind versus trying to reinvent every possible layer. And that's why, cloud as a vehicle, that's why large language models as a vehicle. Getting to the outcomes at a faster pace is the way to think about it.
So really enjoyed the conversation. Thank you so much for sharing your thoughts and your guidance around how people should be thinking about data and the strategy around it in service of the business value that they need to deliver. And Chris, thanks for having us on the show.
Yeah, great to have this opportunity to talk about. Thanks everyone for coming to another data driven podcast. Thank you, [00:39:00] Manish. Thank you, Joe. And please rate and review us your thoughts and stars are really good to have. So thanks everyone for coming and thanks guys. Thanks guys.
Creators and Guests
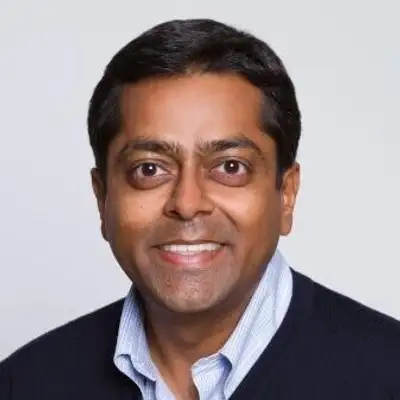
