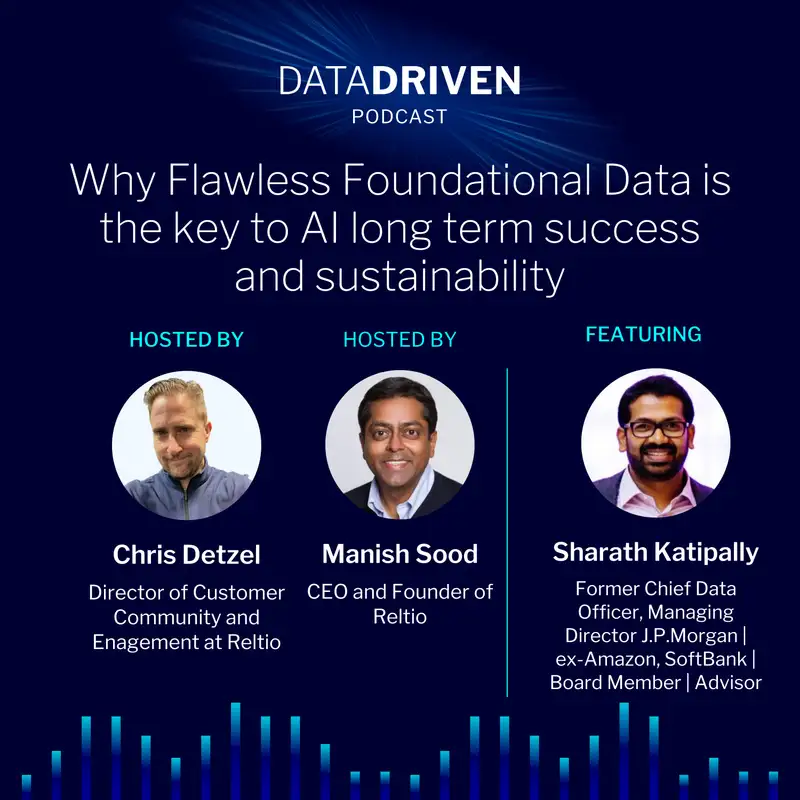
Why Flawless Foundational Data is the key to AI long term success and sustainability
Manish: And the few things top of mind for me as we dive into the conversation are, tied to your career path and journey.
Manish: You've been around data for a long time, you've seen it at different companies in different roles, and it would be just great to understand, a little bit about your career path and how you looked at the various roles and opportunities that you've been a part of at these extremely large organizations.
Manish: So if you can share a little bit of that background, I think everybody is very interested in learning more about
Sharath: it. Let's do it. Thanks, Manish. Firstly pretty excited to be here. If I'm talking about my, if I were to talk about my background, I think I would say I probably rather had a very.
Sharath: non traditional background because once after school, I had a clear choice whether to become a software engineer or do [00:02:00] something with the data. And then back in the day, it was not really so clear to me because of the immigrant visa where I was. I picked up data and got started there.
Sharath: And I think the first decile of my whole career was most about keeping up with the visa and getting excited with the Boutique consulting firms are working for various clients. But my career growth and progression really kicked off in the second style of my career, right? If I put together 18 different clients or customers, I worked for three different locations, various successful leaders.
Sharath: I had an opportunity to share the table with. And I think if I look back it's a great privilege, and I feel that I'm fortunate to see where where I am to look back. I think a couple of key themes jump out to me, right? Why I spend my time first in financial industry and consulting firm.
Sharath: I worked for HSBC and then. tOwards the 2008 when the whole Basel 3, Dodd, Franks and the liquidity [00:03:00] issues have started coming up. That was a time where banks were shrinking. So it was clear for me that I probably have to step out and do something. So I went into one of these startup kind of companies, a reverse logistic company, which is Brightstar.
Sharath: This was later acquired by SoftBank. I was responsible for it. Manage their whole of their information and data stack. It was a global company. It was later taken over by SoftBank. I did various things around. Primarily the business model of this company was around the reverse logistics for, Telecom devices.
Sharath: So there could be access to these cell phones in 2007 and eight with the advent of iPhones, their profit margin just rose and there was a residual value for the reverse return phones and the company being. Their cash cow was reverse logistics. They take the refurbished the phones and then sell sell into either secondary markets or even secondary market countries.
Sharath: It was just a good opportunity. I had an [00:04:00] very horizontal view of right from sales channel, source channel, marketing, sales, and also data business. There was one interesting thing which happened there while we were trying to really work with the, price residual values, price erosion curves, price protection curves, right?
Sharath: This was mainly about how do you really drive incremental revenue and business for this business and how do you compete well with the other companies? And it was all about how do you price your cell phones a dollar less for the secondary market so that you basically get the conversion. In the course, we went with the new sales channels of eBay.
Sharath: Amazon started putting stuff there, grade A, grade B, grade C, grade D phones. And long story short, that particular relationship built my connection with Amazon. And things fast forward, I found myself with an offer with Amazon. And then there was a confidential project in place. And I had an opportunity to work and work for one of the best [00:05:00] bosses of my career.
Sharath: It was an Amazon Go. Where we were dealing and we were dealing with vision technology, buyer mentality, personalization at scale, everything around the retail the physical retail which is completely probably diametrically different than online retail, right? Everything around personalization at scale with high value customer, high value actions and so on, And I was there at Amazon, and then I had an opportunity to go and run other concessions business, also primarily defect elimination, everything around the customer experience. During that course in mid of COVID I had this connection and the offer with J. P. Morgan to go really transition where I was with Amazon, the way I would encapsulate Amazon's experience was.
Sharath: High touch, low margins because of the B to C because of the consumer nature, right? And the evolution into the institutional banking, which is JP Morgan's investment bank, was high touch, high [00:06:00] value, high yield business, right? Which is a B to B institutional business. I thought this shift is good. And because of my pedigree into banking financials it was not daunting, and I was pretty excited to go and really make that shift right at J.
Sharath: P. Morgan. Again, if you look at J. P. Morgan, it's one of a very unique place. There are not many players like that such huge bank with such a reputation, they have mounds and mounds of data. And that is what I got excited. They have everything around. Relationships, trades, client, right?
Sharath: And this is huge. And not everyone in that industry really has all these three or four categories of data. So I was responsible for running the chief data office for capital markets business, which is a very successful business, right? Everything around data production creating a data architecture, reference data, right?
Sharath: And then data products, commercialization, experimentation. This is [00:07:00] all part of the scope. And I if I look back, it is such a great experience to really look at the B to C and into a dive into a B to B with very different business leaders. A tech transition from a tech to a non tech business also, but the non tech is not any more relevant because the banks are rapidly changing.
Sharath: They understand where they have to be and how they have to really upgrade. So we're talking about completely a different set of world. Again, as I said, if I look back pretty pretty thankful and appreciative about how my just carrier unfolded. And I'm looking forward to the next next part of this right now.
Sharath: What I'm doing is helping a couple of my very good friends who are all in the CXO roles or good connections, professional connections doing advisory work across the agencies, includes administration and also portfolio and the FMCC businesses. That is wonderful,
Manish: Sharath. What a phenomenal.
Manish: Career path and journey that you have been [00:08:00] on. And what a great set of companies that you've been a part of. So you're just thinking about that for a second. If you look at Amazon versus J. P. Morgan, Amazon, I can't believe that I'm saying this, but, it's just a couple of decades old you go back 30 years and that's when the business started versus somebody something like J.
Manish: P. Morgan, Has been around for more than a century. sO when you look at, both of these businesses coming in from very different backgrounds in terms of their own evolution, what were some of the key differences that you saw between how these businesses operate or what was the thought process, that was evolving.
Manish: In both of these cultures and companies,
Sharath: I think the very notion of what you said of the tenure tenure of their existence, just just as the is the crux of our discussion. Also, one relatively a very tech product [00:09:00] engineering mentality tech company, which has been probably for the last two decades.
Sharath: The other one has gone through, which is like the fortress Been there for a century after multi acquisitions, multiple acquisitions, right? It was a difference in mentality also, right? And let me allow me to just segment this across multiple lenses. I would say one, the way the business is done specifically in our lens of data.
Sharath: It is, there's a certain way of centralized versus decentralized structuring. That is what I would encapsulate this as, right? Within the tech is more centralized tech is more decentralized. I'm sorry, right? Engineering driven or product driven, where in some facets, engineering is the one which takes the first leap and then product really catches, then product takes the one it can go on with that, right?
Sharath: But if you look at the Traditional investment banking, consumer banking or any wealth management. Also, it is very siloed, [00:10:00] but very centralized. I'm saying this not by taking sides, but this is this has been done. I think They have I've been in the organizations, I've checked a lot of folks around in the industry while I was new.
Sharath: There's a lot of pivot which happens from centralized to decentralized, right? Now, program,
product, design engineering, data, AI. It is all, it could be decentralized in some sense, but again, it is at the enterprise level, right? Because you have so many lines of businesses. It all boils down to two things.
Sharath: Centralized decentralized really really defines the bass for action. The amount of velocity what you can input in the business, right? That is one. Second, just with the nature of day being the industry being in business for so long. The biz tech mentality is something which could be very different.
Sharath: That means in the tech companies, you will see a lot more tech users, super users, ultra [00:11:00] users who are very hand, who are very, who have a very savvy hand and they could go and start deep diving. When you look at the financial sector just because of the operations, their care their core business mentality, right?
Sharath: Not every business leader is very. Tech savvy, data could be data savvy, but not tech savvy. That means they're still relying on analytical engines, vending engines who on a daily basis or a monthly basis, they have to start coming up with any kind of dashboards or any kind of vending technology.
Sharath: So that probably is a crooks of that. And then overall, the culture of iteration, right? The non disruptive mindset or cannibalizing mindset. The self cannibalizing tech mindset, which is what is welcomed and very intentional in tech. Sometimes it is. I did see that is not very prevalent in that industry.
Manish: So as a data leader, Sharad, when you think about the two different styles [00:12:00] are methods of how these organizations work, what were the skills or the areas where you have to think differently when making a transition from one to the other, both are at scale businesses, both are leaders in their own respective industries.
Manish: And there's something about their businesses that they're getting right, but at the same time, there is an opportunity for transformation in each one of these businesses. So how did you grapple with that as a data
Sharath: leader? It was an interesting, inflection also, right? At the tech industry. Because I had some decent level of foresight into how the financial industry works.
Sharath: I could see that what really is a normalizing thing in terms of velocity, the speed of output or go to market in both industries comes down to audit compliance and regulatory, right? If you look at overall, the regulatory wave in a value chain of regulatory wave, the e com, [00:13:00] the social commerce, the ad tech, the martech, then comes the financial institutions.
Sharath: Either could be consumer banking. And on top of the value chain is investment banking HIPAA, which is biotech, and then the space tech, right? If you inverse all these triangle, what are the value chain, what I was mentioning, right? My shift was from probably I could not say in terms of regulatory, the PII, the respect for PII and the EU regulation.
Sharath: What? What? What we have to really live with that does not change. But on a day to day basis, what is the go to market speed? What defines in tech? Is completely different in what defines and in financial industry, because constantly any kind of GTM or go to market, you have to think through the lens of, is it regulatory compliant?
Sharath: Do we have? Are we protecting the business? Are we protecting the client interest to the customer interest in every facet of it? I think that was one of the Challenge also, which I grappled [00:14:00] because it was very much a product and engineering and commercialization leader, any kind of go to market strategy has to go through this.
Sharath: And that is where it just dawned upon me. That a traditional way of audit compliance regulatory probably is not going to suit. And we're going to probably I think in the later part of the podcast, I think we're going to talk very much about AI at some point. I'm sure this is where my fears just unfold because.
Sharath: When the institutions which have been sitting on this mounds and mounds of either could call us referential data, master data or unwielded foundational data, if that is not accurate or correct, right? And with the AI wave, which is taking you from a very left spectrum to the very right spectrum of 10 into hundreds, right?
Sharath: You're moving from 10 to 20 miles to a hundred miles. It is still being subjugated on all [00:15:00] the foundation data. So from a product standpoint, I was able to really make a difference about thinking the culture influencing. But certainly I did grapple with regulatory audit and compliance that wing, and it just felt like I started interviewing a lot of SAS based companies who could come and solve this problem, not with operation heaviness, but with SAS fundamentals.
Sharath: Somebody who really operationalized this created this as a SAS. So I think that, again, in the interest of data and the data strategy this, these were the two big diametrical things which I was dealing with. Sure.
Manish: When we talk about data and, the way you talked about it, it's foundational, especially when it comes to core data that you're running your business on, customer products, supplier type of information, or when we talk about it in the financial services.
Manish: Industry context than it is client or securities, type of information that you're thinking about [00:16:00] having as a foundational core asset that you can reuse across multiple touch points or impact multiple business objectives. But at the same time, the traditional way of thinking has been.
Manish: Building a separate solution, a separate silo each time and at the same time, we talk about centralized versus decentralized. So if we put all of that on one side and think about, what is needed as a foundational capability without going into the centralized versus decentralized argument, what would be some of those areas that you would think about as being core and foundational from a data perspective?
Manish: Because you're right. Thank you. First of all, no discussion is going to be complete without the mention or, touching upon the eye. But I also has a reliance on or data assets being organized in the right manner. So would love your thoughts on, how you think about it.
Sharath: I would take the liberty of course correcting one [00:17:00] of your final anecdotes, right? AI is dependent upon AI is largely dependent upon the foundational data, right? That is your anecdote. For me, it just translates to AI cannot be independent of foundational data. It is very much part of it. And that is the thing where business leaders, the technocrats, everyone have to understand that one.
Sharath: And in I will come back and really paint a picture of what I see as the future also, but Just to take it back and taking a step back when I started my career, when I was starting to learn from individuals like you probably have run bigger enterprises than Knightsbridge was my go to and look up to that organization later was acquired by HP.
Sharath: If you look at what are the paradigm shifts at that point. And from where we came to care came from to where we came to right now was a seismic shift, I would say, [00:18:00] because the four major pillars, what we were dealing with was the four V's, I would call it right, the volume, the variety, the veracity This is what data business was all about.
Sharath: Hey, are we real time? Hey, what kind of heterogeneous data are we dealing with? What kind of variety of problems do we have? And what is the amounts of data we're talking about? Now, if you look at this one, the hyperscalers, the cloud companies, the data cloud companies, everyone has really solved those four problems.
Sharath: Velocity is not a problem. Volume is not a problem, right? But the Transcribed by https: otter. ai The conversation has shifted from the four V's to what I would say is the four P's now, right? That is prediction, precision, productivity, and personalization. And I would extend it up and say personalization at scale.
Sharath: So to your question, when we are dropping, when we're not really grappling with veracity and variety of problems, foundation data still goes through that. So [00:19:00] that needs to be very independent. So a centralized, a fastly paced teams which are very much cited upon solving the foundational problems need to be done very fastly and very precisely.
Sharath: That also means investing, I think. In modern day compliance companies or modern day tool stack companies, and this is where it gets really busy because no matter what, if the foundation data is not accurate, your fortune is still being running on the rails, which are broken. And very interestingly the one way to go about it is it data business is never going to be an accurate business.
Sharath: Or if I come in and you come in, which is the reason CDO jobs are probably the most difficult office to run out there, right? Gartner basically said in 2019 the average tenure is 3. 2. And from then up until now, it [00:20:00] has probably gone 300 basis points down now, right? Again to summarize on this one.
Sharath: What is what comes to be? The core is three aspects. How do you really Curate and unify the reference data and which can be a continuous C. I. C. D. Kind of architecture, which has to be complete and continuous as and when the business is evolving. That means your foundational data is constantly changing.
Sharath: So there needs to be constant investments around that. Second, the data product innovations are very much reliant on the foundation data. And whenever you go through the major major Acquisitions this will come back into picture, right? And finally, I think the volume and the sorry, the velocity and the volume is not a part of the question anymore.
Sharath: So only if we take the 1st 2 into consideration, I think there will be a lot more of success and. In fact, companies will be protecting themselves from a lot of fines is what I'm going to stretch and say which probably I'll be talking when the AI [00:21:00] discussion comes in.
Manish: And, when you think about that, the, you talked about the curation and the unification of the data, but the reason why we would look at something like that across every business, not just in financial services.
Manish: Because we want to impact the business outcomes with the same set of interoperable data asset that is core and foundational. So how do you, in, in these organizations, how did you think about the consumption of the data and, how did you piece together a sequence or the path forward in terms of how to drive the reuse of the core curated?
Manish: data asset that you had started building
Sharath: on? I think I would say, there's a, there's an anecdote which I constantly use and which even though it's such a old one, it still really holds true to this date. Data rich is [00:22:00] oftentimes information poor, right? Only when you really invest in data strategies that can be easier.
Sharath: Now what happened is there is an asymmetry between compute and storage. The storage is getting. The data is getting exponentially large because everything is spitting out and vending out data and compute is getting incrementally cheaper. Now this is like a perfect storm between compute and storage and this asymmetry.
Sharath: Is going to drive a lot of hallucinations coming into the pieces, right? And now, specifically, how did we think about it? And what was my my overall take is focus on the foundational, protect the business from audit and compliance. Constantly keep the continuous improvements on data commercializations, meaning test out the rapid prototyping.
Sharath: and experimentation as a mindset, right? Experimentation is by virtue of it, you are [00:23:00] 50 percent likely to be failed. So how do you really go about changing the opinion for the business sponsors and stakeholders that experimentation is not a luxury, it's mandated, it judicious. And that is where you will go right, you will learn, you will incrementally innovate on things, right?
Sharath: So that culture really helped us forward, right? Meaning we constantly not only said we took an approach of audit compliance and reference data, which is mandated, but it is oftentimes looked at defensive approach. But while we have a curating of that. Curating that, taking a small amounts, taking small subsets, going on incremental innovations, providing data products, either for clients and customers and being able to look at their usability observability.
Sharath: That really changed a lot of mindset. And the shift is, how do you start from an experimentation mindset? Where [00:24:00] largely your outcomes could be 70 percent unsuccessful to getting them into to the finish line of 50 percent or 500 plus operations. That means when you move the needle from left to even though you could still be around the 50 percent mark, but how do you consistently hit that 50 percent mark?
Sharath: That is where the success was.
Manish: Got it. And Sharath, as talk about the culture of innovation, because every, everything, you're thinking about what is the next set of things that you have to go deliver on and drive incremental and transformational value out of, how are you advising or guiding the teams that you are engaged with on how to combine AI and data strategy together?
Manish: What's being the process there?
Sharath: See I think it just given the amount of time I spent in the industry, it is very clear that data and [00:25:00] I can be separate functions anymore. And if you look back money, let's go a decade back. Then the argument was. Can data and analytics be separate functions?
Sharath: Right? Most times data was separate. Analytics was separate, right? The analytic leader could be somebody who's more coalesced and very much augmented, aligned with business side because once the vending of the data happens around any different application or tools or dashboards, whatever we call, that particular leader is more aligned on the business side.
Sharath: But the data harvesting teams who are the ones who are doing the lift and the shift and the transformation, they completely understand what it takes in order to really manufacture new data sets or really curate new data sets or go and go and really update any kind of data sets. When a business logic is changing, the difference in mentality with the two leaders have always created friction.[00:26:00]
Sharath: Now, finally, we started seeing big C roles, CDAO the data officer and the analytics officer, pretty much one. They can't really exist in isolation anymore. They have to coexist. Now, I changed that paradigm to Data and AI. Everything what you're talking about AI I think it becomes important to talk about this distinction.
Sharath: Let's just go back to the history and I'll come back to your question, right? AI has always, at least in the business stakeholder leaders, right? The business sponsors who have been very busy running their business. A little distant away from technology. And this usually happens in the healthcare and the deep financial institutions.
Sharath: They've never really witnessed what the practical use of AI could be, because in the advent, if we look at where we came at least back in two decades back also, first, it was dimensional modeling relational modeling. Then came everything [00:27:00] around the MPP systems, the massively parallel systems.
Sharath: Then we started looking at Hadoop. Big data, M. L. A. I. And finally, we got into the l. M. S. M. L. models, the large language and the small language models. But it was only with the generative A. I. Whole of the business world and community started having a cognitive interface to react that really changed their whole expectation of what technology can do.
Sharath: Which kind of leapfrogged from somewhere in the 10 percentile range to the 90 percentile
range, and that really increased the push for expectations on it and tech also. Right now, if this is important to understand, because where we are going back is now because of that expectation, the it's or the tech worlds will be under immense pressure to deliver something at a fast scale using the foundation model.
Sharath: But now the foundation models cannot be [00:28:00] curated so easily because the foundation model are built on the data strategies, right? So the answer is, I'm starting to propose that. By all means, data and A. I. Has to be under one umbrella, and it is one responsibility. The application of data of A. I. And commercialization of data products that could be a separate office.
Sharath: And this is what I'm starting to even advise and instruct the companies especially in their two years and three years down the lane strategy. And in terms of
Manish: connecting the technology investments, whether it is AI or data or the combination of those 2 to the business outcomes, any guidance and how people should be looking at that?
Manish: Because again, I feel that if, technology remains disconnected from delivering business outcomes that in itself becomes a big hurdle for these technologies as a starting point.
Sharath: You're right about that where [00:29:00] my, there are two quantum of instructions, what I'm trying to put together one, the CEOs, the ID infrastructures.
Sharath: Head of I. T. Infrastructure. Usually that sits around C. O. Be very wary of infrastructure cost. Because you're currently moving from almost liquid engines to a propane engines where you do not even have a seam. You're not moving at this. You're not comparing oranges to oranges in terms of infrastructure now with the asymmetry between the compute and the storage, the compute becoming so fast or so cheap or the storage again.
Sharath: Both are going that way. If you do not really look at the infrastructure costs. You will be shocked by the end of the year. What really shows up right? And now you can push anything into the foundation model. It's going to take a spin, right? But the questions what are going to be asked with conversational AI or could be any cognitive AI.
Sharath: You have to be careful about the infrastructure [00:30:00] costs. Number one. Number two, what I'm also, in fact, I stretched the truth and I expanded and really worked most of the December to understand what are going to be the downside of hallucinating AI engines, because I've started to see the portfolio companies or other companies, I'm not much into the biotech world, but the other companies.
Sharath: I'm starting to see that 2024 2025, business is going to make some hasty decisions based on Gen AI conclusions. And you're going to ask, the amount of veracity, the amount of truth and fidelity, what we are going to put into the foundation models. And we ask any question and it's going to really give you that confident confident, hallucinated answer.
Sharath: Business decisions are going to be based on that. And I will give you an anecdote and the one of the data point around that, right? If you look at NY times suing open AI, [00:31:00] And the big four, just yesterday, the newswire hit the yesterday, the big four, which is all the big four consulting companies, they're putting away some money for really tackling this problems around misinformation.
Sharath: What is going to come back to, this is going to be very important. So those two themes, watch
out for your infrastructure costs. Second, have a very strong hard guardrail around what are the business decisions you're going to make based on the predictions of the engine because your foundation models are not all that healthy yet.
Sharath: And just do a mapping one on one mapping with what you regulation said and what your foundation models are going to do. It just feels like by 2026, the fines and regulations will be thrice or quadruple in the first two years of first EU mandate what came in. And that,
Manish: by the way, is going to bring the focus on explainability and the traceability back into the data [00:32:00] that is used to make the decisions.
Manish: And
Sharath: you're exactly right. Now explainability. Even observability. Also, this all ties back to where your traceability matrix. What? What are you going to do with the data quality? And how do you really come back to the data foundation? A very good site has to be given to the foundational data still.
Sharath: And that is that has been that has been my team also for all these companies. So as we look at
Manish: the future trends, how do you look at the time horizon to adoption for some of these types of capabilities in a meaningful way? I know that there will be some experiments. I know that there will be a race to trying to get to outcomes, but that race is also going to be fraught with certain failures that you are already alluding to in this process.
Sharath: The way I look at it is one. Just let's have a very hard look at what percentage of code is written by AI engines [00:33:00] starting last year. It already started, right? It's going to be a double digit or a triple digit growth. And I would go and stretch it up and say, AI is not going to replace engineers, but engineers who use AI will replace other engineers.
Sharath: The faster, right? If you look at overall 2024 in the paradigm of 2026 and Gartner research and multiple researchers have talked about this one, right? 26 to 30 percent of the whole code written in 2024 and 2025 will be through AI machines. That means they need a bigger, larger oversight to start working around them.
Sharath: And most of the pretty much the Hallucination again the, when it comes to the question about the Hallucination 2024 will be a big, there will be a big discussions about The AI hallucinations and strong conviction [00:34:00] and confident failed answers. How do we really protect ourselves? So the the questions will be back onto this.
Sharath: But again, on the contrary, there'll be lot of business value, which is driven out of 2024. But not significantly as much as we were expecting, because most of the investment in 2023, which went in. On AI and Gen AI. First, it was a hype cycle. Everyone wanted to be part of it. So 60 to 70 percent of that might not yield the results.
Sharath: But the meaningful yield of 40 percent of that results will start shaping in 2024, right? The bigger the company, it's I would still be cautious about how quickly can you move, especially on the conversational AI and Gen AI. Because the answers what are going to be given still, it is very much based on the foundation models.
Manish: Overall, consciously optimistic and, a large part of our path forward is going to be informed by some of the [00:35:00] failures that we have to pay extra attention to, because I think, this path of adoption of AI is irreversible, but at the same time, How quickly, how fast we move towards that kind of a direction is going to be informed largely by some of the problems that we anticipate and figure out ways to
Sharath: address this very true.
Sharath: And let me also pivot this and ask you a question. Also, you are running this company, which deals a lot with some of the secular growth companies, right? Now, a discussion could be the growth cycle Who does it really benefit? And one, the benefits are pretty obvious, but who really takes the burden of this?
Sharath: CDOs actually take the burden of this because the whole of their focus is going to be back on the CDOs, but how clean your data is, what is the traceability of the data quality is established and how do you protect the business, right? Because at the end of the day, [00:36:00] everyone wants to win the race, but turn around, you're still have to, you have the responsibility to protect the business from any kind of audit compliance and regulation.
Sharath: What is your view? I'm pretty interested to understand your view on this.
Manish: Sure. When we think about this overall equation, there are lots of technology foundational capabilities that are becoming available. AI is one such capability, but at the end of the day, the true value of it lies in.
Manish: How are we going to apply it to driving business faster, better, cheaper? Toward business outcomes, and that's the whole promise that we have to start unlocking out of it. But in order to get there, I think the think of large language models or the small language models, all the innovation that's going on there.
Manish: Even the. The user experience innovation, which made it easily adoptable. By every user, those are infrastructure level innovations that have taken [00:37:00] place. Now, how do we take that foundation of infrastructure level innovations and apply them to solving specific business problems? That's the, area where we have to connect the dots.
Manish: And I think that is going to be very specific to very subject matter specific areas. So that is going to require an expertise or a combination of certain industry, certain vertical, certain problem space that you pick and choose and apply these infrastructure capabilities to get to a faster, better, cheaper type of an answer out of this
Sharath: current agree more with that.
Manish: Yeah, so I know that there is 1 such area where you are involved in applying. There's louisiana. ai that's one of the companies that you're advising and working with. Tell us a little bit about the work that is going on there.
Sharath: Sure see that was an interesting time. Middle of the year there.
Sharath: When I joined the investment bank, one of the [00:38:00] biggest opportunity in the investment banks or anywhere when you look at it, because all the trade desks either could be fixed income trading rates, macro derivatives, anyone, there is always a. Time decay factor. When you're doing investment trades, if you have a seller and on the sell side constantly tries to buy a sell stuff to the buy side.
Sharath: And if the matchmaking does not happen in a quick interval, there is a decay. And that's pretty much the alpha and the delta decays what we talk about, right? A very hyper scale. It happens in the investment banking and If you look at the overall TAM across the investment, there are not many clients.
Sharath: There are X thousands of clients which still have a lot of revenue around moving. So a biggest missed opportunity usually happens when you're trying to do one of the REITs trade or say a macro or derivative trade and within a day's time you have to find the buy side. So you could go to [00:39:00] Japan and it's a global business right across time zones.
Sharath: And that Typically happens not much with assisted technology, but still have very manual dealmaking. So loser exactly comes to that. At least it came into the inception with that thought. So primarily, it is an very much an AI powered intelligent revenue enabling platform. It can be. It can be a very fit for global financial firms and very well, very much will expand beyond global financial firms and
The missed opportunity is what I was trying to tackle.
Sharath: And then Goldman Sachs was using this while I was thinking about this. And then the founder Rohan Docter is a very veteran veteran founder who was in Goldman for about 26 years, then came out and started his own company. And he said, he spoke to me and said, so that if you ever step out and if you feel like engaging with us, I'm happy to.
Sharath: And so when this [00:40:00] happened, when I was thinking about thinking about the next opportunity or taking a break and really coming out, this happened. So I joined them. This This company, Luisa, is very interesting right now, and I'm very excited about where it's going to go because it's going to fill that missed opportunity bucket and incrementally drive the revenue by making matchmaking with AI powered matchmaking and everything around scraping right from strong ROI based ability to trigger the revenue generating ideas.
Sharath: It's an internal product. Eventually, it's an investment grade internal product. We are I'm helping them mostly to run their technical technical deep dives and also be able to share a lot of knowledge. What I have that is the advisory role. What I'm doing. Mahesh is the CTO who runs out of Bangalore is doing a pretty phenomenal job.
Sharath: Very smart. Stella 25. Plus team Rohan runs a team, very lean and mean also that's one of the other impressive thing, which I like about it. [00:41:00] And in today's world, capital efficiency is the hallmark of excellence. If you don't, if you don't know how to use the capital capital effectively, I don't think there's not a lot of upside, but yeah, for
Manish: any business, for any business out there, small, medium or large.
Manish: Capital efficiency or being great stewards of capital is a key thing that everybody has to pay attention to. Yeah,
Sharath: and it was even more important getting into 2023 because that particular nerve was just was so important. 2020 and 21, there was a lot of liquidity in the market.
Sharath: Coming into 23, it wasn't that. So that is where Ron really, I got gravitated to this idea and I said, yes, this is something where I want to be involved. So we Louisa has a long way to go, but the pitches are happening. Clients are being very happy. They're receptive about this. And yeah, it's a good story.
Sharath: Yeah,
Manish: and a great example of how taking, something generic like AI, applying it to a specific business [00:42:00] problem and creating value out of it which is a pattern that we are going to see more and more of.
Sharath: And if you, very true, very well said also Manish, if you look at it, banks, financial industries, they have solved a lot of interesting problems.
Sharath: And if they can think beyond and see. What really worked here actually works everywhere, and that's how Amazon really thought about AWS. And now that's how every other cloud company has come into inception. So I'm sure in the coming years, banks are going to start out with this new thing about really white labeling their services and spinning off entities.
Sharath: And Luisa is exactly one of those things. It just worked at Goldman. So it will work everywhere. And that's the belief and conviction what Rohan has, and he has come out with that.
Manish: Sure. Thank you so much for your time today. It was great to talk to you about these topics, especially ranging from your experience and data how you have [00:43:00] used it as a strategic asset with this constant curation type of a model and continuously improving the quality of information that is going to be leverage leveraged as a foundational bedrock.
Manish: Of driving business outcomes, and especially as we look at the eye, the next frontier again, the dependency on data is going to be key, and everybody has to think about how to get that right. Thank you for sharing your insights and your experience. With us I'm sure everybody will enjoy listening to this episode.
Sharath: couldn't be more happier to be here, Manish. It's always good to have a chat with you. I know I've been following and looking at your work. I'm pretty impressed with that. And yeah good luck with this data podcast also. It's a, it is the right timing to talk about these topics and really open up and share knowledge.
Sharath: Because. What we are really moving from is on a quick scale. We are just, we are moving from very left spectrum to the very right [00:44:00] spectrum at a huge scale. And I know that this podcast, you're going to invite good leaders who are going to divulge a lot of meaningful information and the community will will get benefited with that.
Creators and Guests
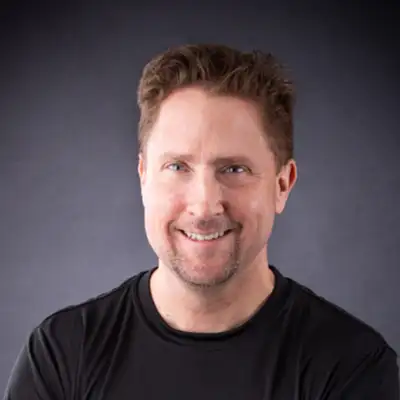
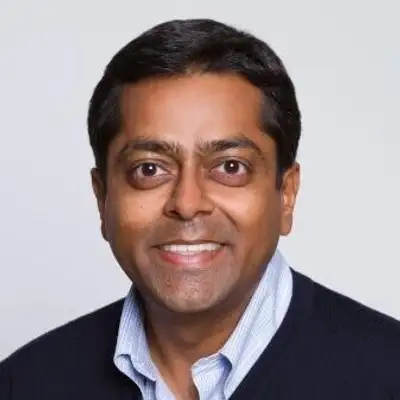
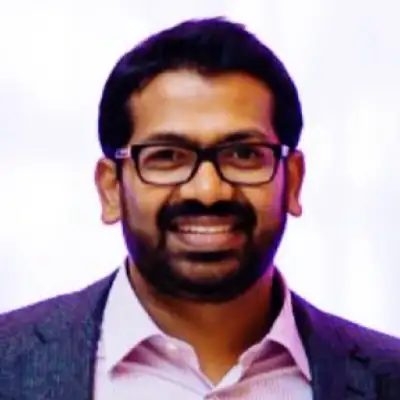