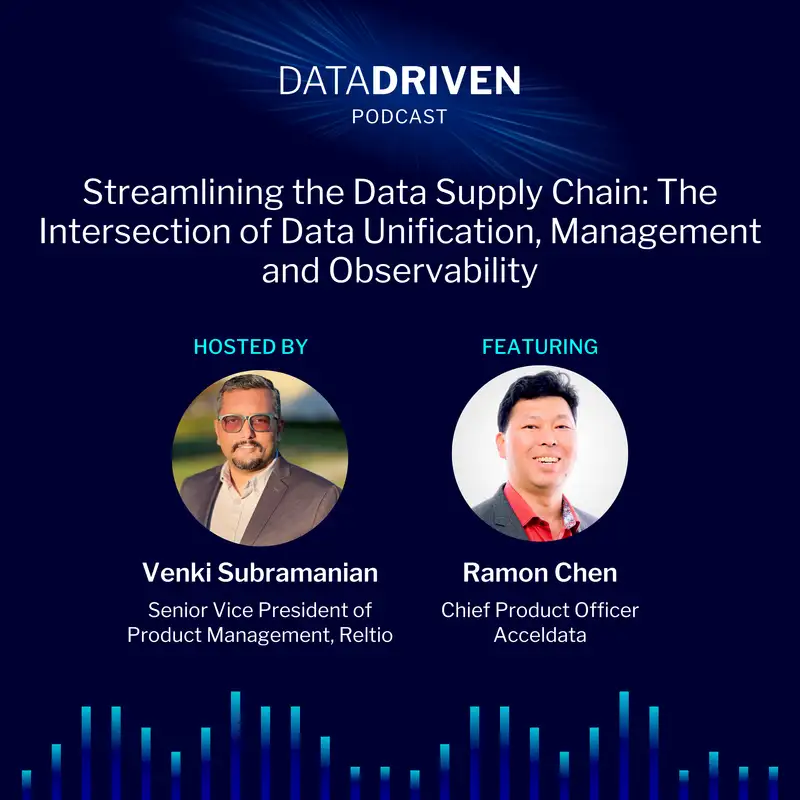
Streamlining the Data Supply Chain: The Intersection of Data Unification, Management and Observability
(0:06 - 0:53)
Welcome everyone. Welcome to yet another edition of our data-driven podcast. My name is Venki Subramanian.
I'm the Senior Vice President of Product Management here at Reltio. And today I have the pleasure of inviting a special guest here to the show with us, Ramon Chen. Ramon is a Chief Product Officer at Acceldata.
In just a minute, Ramon, I'll hand it over to you to give us a more detailed introduction of yourself. But before that, today's show is titled Enhancing Data Reliability and Operational Efficiency through Observability, Data Unification and Management Solutions. And in today's show, we will be talking about the critical role of data observability, data unification and management in enhancing operational efficiency and reliability.
(0:54 - 1:54)
And we will talk about some of the recent innovations and future trends in the data landscape, offering valuable insights and practical examples of overcoming common challenges in complex data environments. Ramon, I know you quite well. For the rest of the audience, would you mind telling us a little bit more about your role and your journey so far?
Ramon:
Thanks, Venki.
Yeah, I'm very excited to be on this podcast. Thanks for inviting me. Yeah, I've been in the business of data management analytics and now AI for many, many years, too many to mention.
But prior to that, as you know, I was the CMO and CPO for many years at Reltio. So it's a bit of a coming home for me in terms of having this podcast. And today, I'm the Chief Product Officer at Acceldata, and we're the data observability platform for many of the leading enterprises across the globe.
Venki:
(1:56 - 3:52)
Great, great. And again, Ramon, like I said, I know you quite well. I've learned a lot from you during your time at Reltio.
And it's great to continue our collaboration in the same space in two different companies. And in today's conversation, I hope that we can drive a little deeper into data observability and the role that it plays in enhancing data reliability and share some of your experience and learning with our audience as well. So maybe first, to start with, can you explain the concept of data observability and why it has become essential in today's data-driven world?
Ramon:
Yeah, absolutely.
So for those of you who've heard the term observability, it comes in many flavors, but the origination of the term was from application performance monitoring. And famous and public companies like Datadog, Splunk, since acquired by Cisco, and New Relic, all found a big gap. And that gap was that applications needed continuous monitoring and observability in order to maintain integrity, performance, efficiency, uptime.
And that became an essential element for running a business. And reliability was a big factor with that. And today these public companies are worth multi-billions of dollars. And it's a very, very known set of tools and capabilities that people acquire.
Back in 2018, the founders of Acceldata started a company to do the same, but focused on data. Because data itself within pipelines and within databases and repositories didn't get the same treatment and data engineers didn't really have the same tools to do their diagnosis when something went wrong.
And many things could go wrong, right? As we all know in the data supply chain. So that's the idea. But if I were to sum it up very briefly in one word, it's about visibility.
(3:54 - 4:33)
Today, most companies with all of their pipelines and tools are flying blind, and they don't know exactly how their data is moving through their enterprise and they need visibility. And that's what data observability provides.
Venki:
Absolutely.
Yeah. You mentioned that Acceldata is today the observability platform for many large enterprises. What kind of conversations Ramon do you typically have with companies these days and what is top of mind for your customers and what has changed in recent years?
Ramon:
Yeah.
So let's start with things that never changed like death taxes and such, right? Three things. People want to make money. They want to save money, and they want to stay out of jail.
(4:34 - 6:06)
It's really one of those three things that you're focused on. So generate more revenue, be more conscious of cost and optimize efficiency, operating efficiency, and then deal with any compliance and regulations that you might get fined for.
So in order to do that, you need to first understand how your data is delivering the insights and the information for your dashboards to your end-business users, or if you're using now increasingly Gen AI and AI and ML, and also in the context of our discussion today, how that data is going to be supplied to products like Reltio for Data Unification and the old adage, use case of master data management. How can you use better data quality to help master data management get even better?
Venki:
Right, right. And I love the three things you said, make money, save money, stay out of jail. I mean, from Reltio's perspective also, our conversations are very similar with customers.
It's all about how do we enable you to drive growth? How do we enable you to improve your efficiency and then improve risk and compliance and data acting as a fuel for all of them? How do we enable you to have the right data, right? So I think a lot of what we are trying to solve for our customers are similar in nature. We're looking at it as just like you said, you're looking at it from a data observability point of view. How do you provide that continuous visibility? And we are looking at it from that unified view of the data and improve data quality.
(6:08 - 14:18)
What are some of the common challenges organizations face in managing and observing data across complex environments?
Ramon:
Yeah, I think this is a great question. And this is again why the company was founded, right? Back in the big data days, our founders were dealing with the big data revolution, in and around about 2018, where Hadoop was a big thing. But those on-prem machines all had efficiency issues, being able to optimize for how much you'd want to spend in order to process the data.
And the volumes keep going up, right? And it's common for people to deal in petabytes these days, not just terabytes. And the modes of data, streaming, unstructured, structured, and it's not stopping, right? Gen AI is just making this worse and worse and worse. And people creating new data pipelines, right? To be able to move data from one place to another, to deliver it to those target systems.
But there are thousands of thousands of pipelines that have already been built. Some dating back 10 to 20 years, no less. They still run critical operations in enterprises.
And again, there's no visibility. People cannot see. They don't know why tools are being used.
Somebody puts a tool in place or a repository. They left the company. It's still working, so they leave it there.
But do you really need it? Is it costing you money? Is there a more efficient way? That's fundamentally it, right? Deliver data on time as promised of high quality to not just the end user, but to data unification tools like Reltio so that MDM can be better. Right.
Venki:
And I think that common theme about the sprawl in the data landscape, that definitely is a top of mind priority for many customers.
The reason why there is more investment in data unification, data observability is because there are way too many data pipelines because you've got more source systems than ever before, more consumers of data than ever before. And while you're moving data back and forth, you need to understand what data is being used, what data is needed. And that observability as a capability of model, I think that totally makes sense.
It also leads me to my next question about the synergies that you see between data unification and management and data observability. You already touched upon some of those things, but can you dive a little bit deeper into why it is so synergistic between these two sets of capabilities?
Ramon:
Yeah, absolutely. So the fact that we all deal with data quality, right? So data unification and what Reltio does is to create a single source of truth, and a golden record or the best version and contextual representation of the information for the right audience, right? Whether that's MDM, customer 360, identity resolution, the quality of the data that feeds as sources into something like a Reltio makes a difference, right?
As you know, the ability for auto merging and matching and the number of manual matches will largely depend on the supply of the data from the different sources that come in.
So the synergy between data observability and data unification and Reltio master data management is obvious to me, but shifting left is a concept that is widely being spoken about, meaning that by the time the data comes from a source into Reltio, it may be a little bit too late to significantly impact the efficiency of what Reltio needs to do its best work.
So something like an Acceldata is able to introspect high volumes of data coming in from data suppliers, let's say, and be able to detect that, hey, look, this data looks stale to me because I'm expecting these fields to be updated and it wasn't. Or it can also intercept the fact that, hey, there's a new column here. Well, I'm not expecting this. I can't pass this downstream into the Reltio system. The Reltio system is going to need a logical data model change for 360.
So I can't afford to send this through. It might break the feed and then everybody's in trouble because everything gets suspended. So those are just a couple of examples.
But the goal really here, if I could give you some pragmatic examples of how this might improve and what our joint customers are thinking about, as you know, Venki, one of the challenges for data stewards is there's not enough of them. Because no matter how good the technology is with unification, there's always manual introspection. And that's why data stewards exist.
So how does the data steward diagnose and decide on the manual match in a faster time frame? Well, they have to pick up the phone sometimes and speak to people. But the first step is, what's the data that's being fed in and where did it come from? And is there a problem with that data?
So having data observability as part of the flow will give them immediate diagnosis and immediate ability to request that something be fixed, whether it's from a data supplier or whether a data engineer needs to go correct a pipeline for them so that there's not time wasted. So they would file a ticket and then the data engineer would get it.
And the data engineer themselves would not know where to start because they wouldn't know the goal, the consequences, and all the sources. So speeding up that process is number one.
Number two, very simple one, is does the data that comes out of the data unification process benefit as intended the target systems like CRM, like Salesforce, for instance? And did it even make it there? There's a concept called data reconciliation inherent in data observability that checks that the source distributed the data to its target as intended in the right and timely fashion.
And then the final element is the holy grail, right? We all want to know, are we doing the right things to benefit the business and are they getting the right value out of it? We all want to know that we're not doing busy work, and data observability will provide that visibility. Right?
Venki:
I think that's a good model.
So if you look at a left to right orientation, you've got your different source systems feeding data into a unified data layer, like a data unification engine or a master data management customer 360 kind of an engine. And that there are consuming systems on the right side that are consuming data from there. So your point is, how can you insert data observability before the data from sources are fed into that data unification or the master data or the customer 360 layer so that the right data makes it to those systems and reduce the effort for data stewards to understand or remediate discrepancies or quality issues thereby providing those insights through observability.
And then on the right side of that, as you look at the consumers of data, again, use observability capabilities to understand the right data getting to the right systems and getting consumed. And then the third aspect is the value of data consumption itself. Are you consuming the right data in the right places in the right manner?
Ramon:
That's exactly it.
That's exactly it. It's the full visibility to the supply chain, the pre-data entry point source data into something like Reltio is hugely beneficial for Reltio. It'll speed up resolution for manual matches, exactly as you said.
Yeah. To me, the other thing I would say is figuring out why we are doing this, right? I think that's the reason I joined Acceldata. I've been in MDM and analytics databases all my career, and I've really been somewhat embarrassed personally that I can't answer the question.
(14:18 - 15:43)
If you invest X in this technology as part of the IT engine, does the business really benefit? And are we doing the right things? Cataloging is a classic case. Right now, today, I'll tell you that companies are fed up cataloging for no reason, right? Yes, there was a reason to do it for GDPR and compliance, and it's very much a defensive measure, but catalog data just sits there statically. It's not being used in the flow of pipelines and to make business operational decisions, and it's a waste.
So there's a lot of waste out there, and data observability aims to help every single tool in the continuum, not just the data unification tools.
Venki:
Right, right. And I know we have talked about it in other conversations, Ramon, about the role of active metadata and how that is foundational.
Do you want to touch upon that a little bit? I think you mentioned it, but maybe-
Ramon:
Yeah, so some quick definitions, right? Active metadata is a very important concept. You'll hear a lot about it today. What does that mean? So metadata is data about data, right? Every single different kinds of metadata, we don't have time to describe all of the categories right now, but a simple example is column definition, it's type, it's field, it's size, it's the volume of the data itself, the repeated values, the most frequent occurrences, and those types of things.
(15:44 - 17:45)
But that information would be considered passive if it's just sitting within one data source. Active metadata is taking that data, combining it with other metadata, and then taking actions, recommendations on it. A simple example in real life is a thermometer, right? If you have a thermometer and it just tells you the temperature, that's passive and using the temperature as metadata.
Active metadata is a thermostat because it also tells you the temperature, but it also senses the external conditions, which is another piece of metadata, and then it makes and takes actions by turning on your heat or turning on your air conditioning. That concept as applied to the data supply chain is exactly this. Data observability gathers metadata from a variety of data stores, pipelines, and sources, as well as whatever data unification tools can provide and catalogs.
And then it makes operational decisions, like a thermostat would and provides recommendations to say, hey, you should possibly not focus on this data. It's not interesting, nobody's using it. Hey, by the way, it's costing you a heck of a lot of money to deliver this via Snowflake and Databricks.
There's better ways of doing it because I can see the metadata that says you've over-provisioned your size of your data warehouses. So in summary, that's what it is.
Venki:
Great.
Right. Yeah. So we touched upon different aspects or different parts of the problem that companies like Acceldata are solving.
From a definition perspective, Ramon, we touched upon, we talked about observability, active metadata. Are there specific pillars that we need to understand about data observability?
Ramon:
Yes, that's a great question. So Gartner has just published the Market Guide to Data Observability.
It's the first publication of this type from Gartner. It's available, just a quick plug, on the Acceldata website for free. So you can download that guide.
(17:46 - 18:28)
Gartner defines data observability in five categories or key pillars.
The first one is observing and ensuring the reliability of data and the content of the data. So the quality, the nulls, the values, the information.
The second is the data pipeline. It's the mode of delivery. Are pipelines breaking? Are they delivering information with the right throughput? What's the cadence of the information that's being delivered? And so on and so forth. So that's the pipeline and the vehicle. And then together with that, there's lots of things like we previously discussed, like schema drift and those types of detections. So those are the first two pillars.
(18:28 - 22:10)
The third one is infrastructure and compute. So how much processing is it costing to do all of these things to get something from A to B? What are the tools, like data unification tools, Databricks, Snowflake, all manner, your interim repositories, all what's being used and are you using it in the most efficient way? And is it being efficient? And then that's the third.
The fourth is once you understand that, how can you then take that cost and map it back to the business? How can you tell the business users, as an example, if you're running Snowflake queries for a marketing campaign, how much is it actually costing you, and how can you financially chargeback or allocate to those departments so that everybody is aware that none of this is free. You should be more conscious when you run or spam multiple queries all the time.
And then the final one is helping users be more efficient. Once you can show people what they're doing costs money and you can show them that a Select * query is a very bad thing to do, they get more intelligent and better trained and informed, then your usage and utilization will be more optimal.
So those are the five pillars, but more pillars are coming. AI and ML observability is going to become a thing and we're already ahead of that game at Acceldata, but those are the five.
Venki:
Okay.
I think that is really helpful because I think in today's topic about reliability of data and how data unification and observability all works together, it's really interesting the way you laid out those five pillars that Gartner proposes, how each one of them, in conjunction with the unified data that Reltio kind of systems produces, provides the end-to-end visibility about what kind of data exists, what is the unified data, how is it getting consumed? And then the aspect that you mentioned around cost, visibility into cost and the chargeback capabilities, that also then helps organizations tie that directly to the value they're delivering to business and then be able to attribute cost to that.
Ramon:
Yeah. Yeah. The usage is important.
One of the final pillars is usage, right? Which is, can I look at a dashboard and see how often that dashboard is being used by the business, how often it's looked at? That gives a sense on how important that information on that dashboard is. Then if you decompose what fields and tables contribute to that dashboard and work your way backwards, you can then see how critical the things that you do to that data on the run-up to data unification means. So that end-to-end visibility just doesn't exist today.
And I think once you turn on data observability, all of a sudden things just come to light that make everything more efficient, everything more cost-effective and better for everybody.
Venki:
Right. Ramon, you mentioned this a little while before, modern trends in data observability, how AI is changing the landscape and how AI is also probably creating new requirements for observability and data itself in general.
Tell me a little bit more about the role of AI and what is changing in this landscape.
Ramon:
Yeah, there's multiple ways to think about this. The first one is the obvious one, which is that all vendors, relatively included, are injecting AI capabilities or have injected AI capabilities into the platform to make the process of data unification more efficient.
(22:11 - 22:39)
No difference here at Acceldata. We acquired a company last year and we very rapidly incorporated AI capabilities, copilot capabilities into our platform. I like to always think of AI, not necessarily artificial intelligence, but sort of augmented intelligence in terms of helping automate functions that human beings would do in the course of data management.
(22:39 - 25:14)
They're not going to autonomously make decisions just yet, so there's always human in the loop. But gen AI and AI and machine learning is helping speed up the understanding of how to go about deploying rules and policies, which are sort of the cornerstone of keeping track of various touch points within the data supply chain. And anomaly detection is a term that you'll hear a lot these days, which in essence, it's really just finding patterns of data and usage, and that will help with faster root cause analysis and resolution, but also be able to start proactively detecting problems.
As an example, if you see that a threshold of throughput and pipeline has abnormally spiked, it might be an indicator for you to dial up the resourcing and capacity of that pipeline if it's not dynamically scalable. And that's not something that's in place today. It's a very manual process and very reactive.
Venki:
I like your definition of AI as augmented intelligence, not just artificial intelligence. To that point, even some of the things that we have done at LTO to infuse AI more and more into the platform. First and foremost, we started with working back from what are we going to try and solve? It's not for AI for the sake of AI, it's really about how do we deliver more value faster? And then what are the augmentation within the existing capabilities we can do with the applications of AI and automation that is driven by AI? And we also see in the same space demand that AI is generating a lot more data than before, AI is also going to consume a lot more data than before, and that requires more maturity and data management capabilities across the board.
A couple of other things in the remaining time that we have here today. What trends do you foresee in future of data management, unification, observability, and how should data leaders really think about the capabilities that they need to be prepared for?
Ramon:
Yeah, so I really think that data observability, again, being very passionate about this, having spent 30 years in all of these different disciplines and MDM at Reltio for seven. I'm super excited because I think the landscape is really radically going to change in data management.
(25:15 - 29:59)
It's not going to be the same old way of thinking about investing in IT and platform and tools. Chief data officers and CIOs are being behooven by the CEO to say, I've got to have more direct correlation to a return on investment based on the amount of money you're asking me to spend on such tools. I talked about data governance.
The old way of doing data governance and data catalog is going the way of the dodo. Nobody's going to stand for, hey, I'm going to catalog it and then I'll figure out what I do with this information afterwards, right? And we talked about the differences between passive and active metadata to have it more operational data governance, right? And data observability starts with that.
In fact, the trend I'm seeing right now is that companies are actually coming to companies like Acceldata that offer data observability to provide that health check to say, what exactly is going on with what I have today? Before I even spend a dime on data governance or any of these other disciplines, let me find out where the problems are.
And let me also find out who's using what data at my business so that I can really plan and develop the right strategies and buy the right tools and use them cost effectively to solve the problems. So it's definitely, people are leaning forward. They're adopting data observability to solve their problems.
But first, just getting an understanding is the key.
Venki:
Right, right. I absolutely love that perspective.
And the similarities that I can see with some of the conversation that I'm having is there is a lot more focus on understanding the value that data delivers. And instead of just moving data back and forth anywhere in the organization in an uncontrolled manner, and then trying to catalog all the things that exist and where, who is doing what, there's a little bit more structured approach that organizations are taking and can and should take to really understand where is the value? How can the right kind of data be delivered or to be utilized for delivering that value? What are the capabilities needed for that? So that requires, again, observability as a key capability, active metadata as a way to understand data and be able to take actions on that data, and then a unified data layer, especially for your foundational data to power that consumption. And in other podcasts and other discussions, we have talked about concepts like data product and how organizations are starting to see or treat data as a product, which basically means any product that you create, you have to measure adoption of the data, you have to deliver iterative value of the data.
And those are some of the principles that are now getting applied to data itself as in the context of data product.
Ramon:
Yep, yep. And without data observability, I challenge anybody to say, how are you going to do this, right? How do you know that the data is going to be of sufficient quality to meet your data product? How would you even create a data fabric without a level of observability to make sure that this thing is instrumented correctly, that you're marshaling and pulling the right data elements in the data fabric? And then how are you maintaining the SLAs on these data products? And is it delivering value, right? So yeah, that's why I'm super excited.
I think data observability is essential. I really, I'm going to lean forward here and say every single company on the planet, regardless of size, needs some form of data observability. Otherwise, you're back to the same old, same old doing things blind and not understanding whether you're achieving the right outcomes at the right cost.
Venki:
Right. Great. Time flies when you're having fun in the conversation and you're learning so much from this discussion, Ramon.
I understand that Acceldata is sponsoring Data Driven 2024 this year, which is our industry conference, the modern data management conference that we put together October 7th to 9th at Orlando. What are you most looking forward to at the conference?
Ramon:
Yeah, I mean, I think that I'm excited we are able to sponsor. I think that the best companies on the planet come to the Reltio Data Driven 24 event.
And they're the most progressive, largest enterprises on the planet. And they all have synergies with data observability. In fact, I was there last year purely as an attendee and the number of conversations that I had with large corporations, that both were really existing Acceldata customers, but also new ones that were interested in data observability was mind-blowing.
So it’s a great opportunity to share experiences and exchange ideas, to continue to move forward to combine data observability and data unification, master data management and beyond. So I’m very excited that we are sponsoring and I hope to see everybody there.
Venki:
Great, great, and thank you for your support and sponsorship. I’m also really looking forward to the conference, it’s going to be a lot of exciting conversations. Once again Ramon, thank you for the time educating us on data observability, and improving data reliability for data unification and management solutions. I look forward to continuing this conversation, and learning from you on future sessions as well.
Creators and Guests
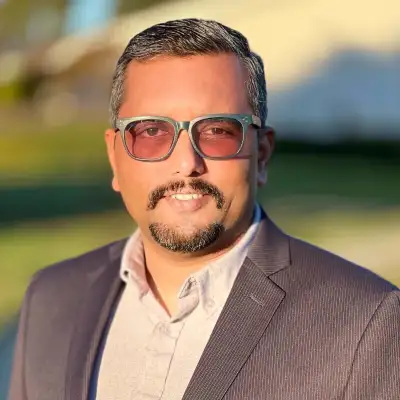