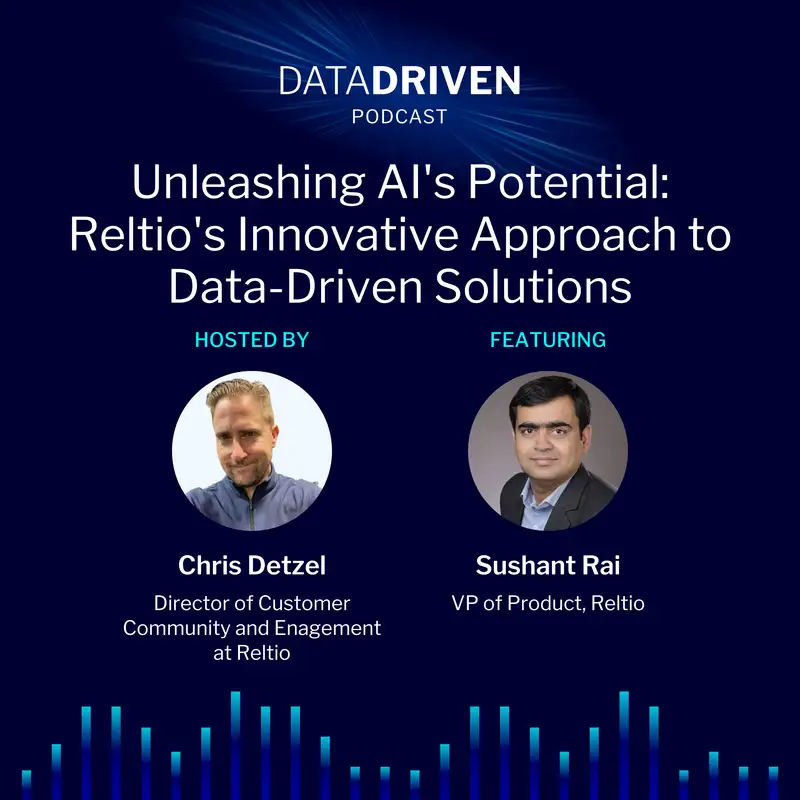
Unleashing AI's Potential: Reltio's Innovative Approach to Data-Driven Solutions
Chris: [00:00:00] All right, welcome to another data driven podcast. I'm Chris Detzel and today we have special guest Sushant Rai. He's the VP of product management at Reltio. Sushant, how are you today?
Sushant: Very well, Chris, how are you doing?
Chris: I'm doing well, man. One of the things that we want to talk about is this idea of the intersection of AI data management and product development.
Chris: Sure, with all the things that AI's A lot of things are being talked about around AI. And so you're one of our experts, you've been in this all day and every day. What do you think Sushant? Good topic today?
Sushant: So it is the most exciting topic these days to talk about. I'd love to have a great conversation.
Sushant: First of all, thanks for having me here. And I'd like to share our journey, what our thoughts are around The enterprise with, market where customers are dealing with this question around the IML in recent days. So it should be a good conversation.
Chris: It's going to be great. And [00:01:00] so before we get started, tell us a little bit about yourself, how long you've been with Reltio, what you do at Reltio, all the, maybe a little bit before what you did, that kind of stuff from a career standpoint.
Sushant: All right. Yeah. So I lead the product management for core data unification platform at Reltio all the IML initiatives and all the new products that we are building here. I've been here for almost four years now, and I must say that it has been a really exciting journey. I've been in the price of software all my career, right?
Sushant: I would say another 18 years in part, different companies before Reltio. But I must say that the scale and impact relative products have on business outcomes for our customers. It's hard to match, right? So I said, as I said, really exciting journey. Really having fun here.
Chris: Good. And the world's changing, so we'll talk about how that is with kind of the as we think about the general trends in AI and like enterprise adoption.
Chris: So let's get started, man. Sure. What are some of the primary drivers for like businesses [00:02:00] adopting AI technologies today and how, before we how do these drivers vary across the different industries. Maybe give us some examples of how AI is being used in some of these industries.
Sushant: Yeah, no, that's a great question. So we have seen that adoption of technology, right? Specifically, is driven by desire to improve efficiency, improve decision making enhance customer experiences and just improve business responsive to in today's increasingly digital and data driven business landscape, right?
Sushant: Let's break them up one by one. The very first problem that everybody is trying to address is how to increase efficiency. In anything we do, and AI can automate tasks, repetitive tasks. Streamline processes, optimize operations, which ultimately leads to increased efficiency and cost savings.
Sushant: It's very important in these macroeconomic times to have these as business goals, right? [00:03:00] If you see from an industry perspective from automating tasks and manufacturing assembly lines to streamlining logistics and retail, AI can free up a lot of human capital and reduce costs for enterprises, right?
Sushant: The second big driver is data driven decision making, right? AI powered analytics provide businesses with valuable insights from large volumes of data that they already have, right? And enables more informed and data driven decision making. There's no difference of data, right? Data was always there and enterprises are generating data like Never before, but synthesizing data and getting insights to make, as I said, data driven decisions is really the key here.
Sushant: Industries like finance, right? Canalyze this data to uncover hidden patterns and optimize risk management, right? Reduce frauds. Or healthcare industries in particular, right? Can personalize treatment and plans for its patient, right? So different applications from the data customers have, or they're generating [00:04:00] on a day to day basis.
Sushant: The 3rd driver I would say is personalizing customer experience, right? I would say AI business
enables businesses to analyze customer data and behavior to deliver personalized experiences and recommendations, right? Through techniques like machine learning and natural language processing. Some of it, what we are also doing at Realtio, companies can understand customer preferences, And dealer products and services do type of targeted marketing campaigns and lead that all of that would lead to improve customer satisfaction and loyalty, right?
Sushant: This is particularly helpful in retail and consumer products industry, right? Retailers are leveraging AI for chatbots and product recommendations based on the past behaviors or purchase patterns, right? Travel companies are using it for personalized itineraries, again, based on past behaviors. Or the demographic data off of their customers.
Sushant: So wide range of applications across the industry for this [00:05:00] particular factor. And finally, I would say a very important thing is business responsiveness, right? But we have seen in our customer landscape as well that the digital world is changing rapidly, right? And adopting AI can provide that competitive edge in today's evolving business landscape.
Sushant: Companies that will harness AI for innovation and differentiation will stay ahead of competitors by introducing new products or services, optimizing their business processes by automation, as I mentioned earlier, and simply by adapting to changing market demands more quickly and efficiently. So this particular part is a factor is going to be applicable across every business and every industry that customers.
Sushant: Are going to our businesses will have to improve their business responsiveness and AI is going to play a major role in that
Chris: Yeah. And, I'll give you one example, as you mentioned sales and marketing and how AI is being used. I've seen this technology that, helps [00:06:00] BDRs with emails.
Chris: BDRs are always emailing prospects and trying to get them to learn about different products within an organization. One idea or one technology that I know of. I won't say the technology but is automating those emails personalizing those emails making it look like seem like it's a real person and then it just Takes a lot of time off those BDRs to go do more, I wouldn't say they'll do more of their tactical kind of day to day type stuff.
Chris: That's just one of many of the examples. So it's changing. And speaking of that, do you think Reltior customers are building some of their own AI models, or are they turning to like off the shelf gen AI?
Sushant: I think it really depends, right? So decision to build. Their own models for JNI or using off the shelf models depends on multiple factors.
Sushant: And. I would say how much weightage these factors have, they differ from business to business, right?
Chris: Yeah. So
Sushant: for example, how personalized or context specific your gen, you want your GNI model to be, [00:07:00] right? Typically off the shelf models lab lack the business context for a particular industry, right?
Sushant: That, that could be a limiting factor. How, data security and privacy compliance across the business is going to affect right using building on their own or deploying their own or using auditions models, right? Do another key factor is what kind of expertise or talent customers already have, right?
Sushant: To build these models from ground up, what is the ease of implementation or maintaining it for long term, right? And what is the time to value? Will they spend six months or 12 years to build a model and then get value out of it and constantly tune it to get the results that they're looking for?
Sushant: So multiple factors that that affect this decision, right? There's no one size fits all model that customers are going to adopt. That's not going to happen, right? Based on requirements, urgency, on time to value and data that they have, customers make this decision. In our experience customers typically look for a hybrid approach that gives them [00:08:00] high time to value data security, right?
Sushant: And specifically, the models are responding to their business context, right? And this is what we are noticing across relative customers as well. We ourself have, provide Gen AI capabilities to our customers, and we focus on all these factors in the capabilities that we provide.
Chris: I've got a big question for you.
Chris: That's coming up and I'm very curious to see how you answer. And then we'll get into the Reltio story, but given that like AI systems rely heavily on data, how do these issues with data quality affect AI outcomes and what steps do you think companies could take to ensure that their data is ready for AI?
Chris: It's a big question.
Sushant: It is but I think. It's a very important one as well, because I believe every business is dealing with it, right? I would say issues with data quality can significantly impact the outcomes and effectiveness. Of AI models in multiple ways, right accuracy, for example, right?[00:09:00]
Sushant: Poor quality data can and will lead directly to inaccurate air predictions or decisions, right? Whatever your insights were expecting, or you are getting poor quality. And give you poor decisions. It's as simple as that, right? If the training data built to used to build or train AI models contains errors or inconsistencies, the resulting models will produce unreliable or misleading results.
Sushant: The second part is like robustness, right? AI models that are trained on low quality data may lack robustness or generalization capability. You can use a small training set data, but when the models will be asked to make predictions to on handle unseen or new data, those predict the quality of those predictions or decisions was going to will be.
Sushant: Will suffer for sure. The third big one that, that that is the customers facing everywhere is bias, right? Data quality issues are just imbalanced data sets or bias sampling can [00:10:00] result in AI models that perpetuate or amplify existing biases present in the data, right? Or this can lead to unfair or discriminatory outcomes.
Sushant: And we have seen examples of this, whether, it's a hiring policy that is discriminating or picking only certain kinds of candidates. And we have seen, or in lending that, you're making unfavorable decisions for, because of a certain bias, this is particularly I would say a dangerous one, right?
Sushant: So investing time and money to train an AI model and look what the data is. It's like wasting your resources, right? Customers will end up with a model that needs to be scrapped and rebuilt entirely. We have to understand a very important thing that data drives AI, right? Data is getting generated at a massive scale across the enterprise.
Sushant: And this, and it is fragmented at the same time, right? This fragmented data has no ownership, is of bad quality and duplicated across the board, right? So the very first thing enterprises need to do is like data unification. It is the first and the most important [00:11:00] step for customers to get high quality data that they need for their AI initiatives.
Sushant: Data needs to be cleansed, enriched, and unified to be fit for any insights or predictions that the customers want to, get out of them, right? Importance of data navigation and complex entire enterprise environment is increasing every day as the AI momentum continues to grow.
Chris: How do you how does Reltio really think about AI and ML?
Chris: What are you, what is it that you guys are doing or thinking about, from say us, really?
Sushant: Yeah, so I would say we have a three part story here, right? I would say we are thinking in three distinct, Categories are three distinct areas and investing in all three at the same time.
Sushant: The way I call it is built for AI, built in AI and built with AI, right? So what is built for AI, right? Solving the data issues we just discovered in our customers. To unlock potential for them, right? We help solve the [00:12:00] gap in data trustworthiness that our customers have, which prevents them from realizing all the AI benefits that they can, right?
Sushant: All the things we just spoke about on how bad data quality affects them, right? So we connect the first party data to models and models to data, right? We provide that. High quality, trusted, unified data in real time for our customers to build models on top of it and then get the accurate predictions and decisions that they need to run their business.
Sushant: The second is building AI within the capabilities, right? So which we call built in AI. Second, we are integrating AI ML into our own products, improve efficiency and productivity for our users, right? Yeah. So our, what we are calling applied AI, which is applying AI across our platform capabilities, that will deliver 10x the value, 10 times the value for our customers than doing it without AI ML, right?
Sushant: So infusing AI in our capabilities. And the third is build with AI, [00:13:00] right? Just like we want to improve productivity and efficiency for our customers, we are also empowering our own teams to build with AI, right? We are using AIML ourselves to build our products, right? For higher productivity, right?
Sushant: Reltians, right? All Reltians use latest tools to increase their own productivity, whether they're in product teams or they're in engineering teams to build these capabilities. We are fully in fully invested in every single area where, wherever we see an opportunity or a problem that we can solve at AI.
Chris: Yeah, and Railtail's been doing AI and ML for a little while, obviously the approach I think probably evolves over time. Can you talk about that evolution from, a few years back till today
Sushant: with Railtail? Yes, definitely. I think you're right? As AIML and the technology has evolved itself, right?
Sushant: I think we constantly need to think in our approach as well. So Relty has been a market leader in data unification, right? For years, right? We integrated [00:14:00] AIML in products a couple of years ago, I would say ahead of the market, but the approach was different at the time. Yeah. What we did was we enabled our customers and provided with capabilities and platform to create their models and for data unification and train them, right?
Sushant: But over time, we realized that the maturity, skillset, and capacity to do these data incentive tasks was not with every customer, right? The time to value for them was quite high, but, and we acknowledge that customers looked up to us to provide prescriptive guidance and product capabilities that will provide their desired business outcomes out of the box, right?
Sushant: So what we have done is. I'd say in the past six months, we have changed our strategy, right? We have created our own technology, which we call Flexible Entity Resolution Network that customers can enable with a single click. And this firm, which will start Suggesting potential matches out of the box, right?
Sushant: PERN stands for Flexible Intuitive Resolution Network. Customers will need, not need to do any [00:15:00] configuration. No data processing is needed from their side. No model creation or training is needed from customers, right? Customers will get instant time to value, right? So that is a shift that we are seeing that, hey, We AI ML models, right?
Sushant: It's not about the technology. It's about the outcomes that customers get out of it Without spending too much time processing data creating models training validating Tuning it right we are going to do it. We are doing it out of the box for our customers.
Chris: So That's interesting. Love that. Can you talk about the time it took for somebody to do that manually versus now it's all automated?
Sushant: Oh, yeah. So if you see let's talk about matching, right? Which is entity resolution, right? On an average, we notice that our customers when they Set up match rules in our engine, natural engine to, to find potential matches, right? Whether two profiles or two customers are same or not, customers would take up to six weeks to set up match rules, then test them against [00:16:00] data to I would say then tune it further and then test it again as data, and then multiple data loads would happen and things like that, right?
Sushant: It would take up to six weeks to get to that. And then tuning will continue. For a very long time. And then this, these matches would generate tens of thousands of potential match pairs for data stewards to review one by one, and then take decision. What our models are now doing is completely taking away that set of time and starting it in an instant. And then the accuracy that we are getting out of a match, of machine learning models are much higher. The ML models are able to find matches that no match was able to find before, right? Because how they are built on large language models and, the technology associated with them.
Sushant: So we are looking at faster time to value, like no set of time, as well as faster processing. Of, of potential matches.
Chris: So like from six weeks or, from six weeks to days to [00:17:00] hours, what's the thinking, two
Sushant: hours, I would say,
Chris: wow, that's crazy. That, that's the example that I love, like that's real life, and this is happening.
Chris: It's only going to get better, from here, so that's. Really cool from an entity resolution standpoint. So you shared some examples, but maybe we can go a little bit deeper. Could you share like a little bit examples of AI and ML features currently with Reltio's product and how do these features enhance user experience and outcomes?
Chris: You mentioned one, is there others?
Sushant: Oh, yeah. So let me take two examples, and I would probably do a double click on the one that I
shared or, just now. So let's talk about that one. It's called flexible entity resolution network, which is fun.
Sushant: It's essentially a large language model powered capability that automatically suggest matches out of the box with much higher accuracy, right? Basically flexibility resolution network. It enables. Instant automated semantic understanding of all entity data, whether it's customers or [00:18:00] persons or individuals or product supplier, right?
Sushant: Any kind of entity that is there one instantly understands it. And, new types of matches that are rule based that rule based approaches cannot find points semantically understands that data and starts working. It's built on, fine tune deep learning models. That use data element for comparisons, produce high quality and high confidence match recommendations or multiple domains, as I mentioned, person organization, a key thing is that these pre trained models incorporate zero short learning.
Sushant: What is zero short learning that customers need not train this machine learning model at all, right? It will start. It will immediately start giving results or make predictions on matches as soon as it is enabled, right? So it increased data team, increases data team productivity, shortens or eliminates pretty much implementation time, right?
Sushant: So that is just [00:19:00] what's out of the box, right? It also is like we are completely data security and compliant for privacy as well. Customer data never leaves the tenant at all. It is always residing in the tenant. Predictions are happening locally, right? We do not send the data to Pyperscaler. We do not send data to for any kind of training or predictions to outside Realtio.
Sushant: There is no training data shared across customers as well, completely secure and private, right? And it has explainability built in for match recommendations of a data store. It wants to know why did phone recommend these two profiles of these two customers to be same? We explainability is built in, right?
Sushant: And some of the key capabilities, right? It has a global impact, right? With natural language process of human 60 human languages. Just taking an example, right? You would write the same name of a person in English and in Japanese. Porn will be able to identify that, Hey, these two are the same person, just the name written in different characters, right?
Sushant: So that, that is the power of porn that we are able to offer to our [00:20:00] customers. That's a breakthrough, right? This is a patent. We have already filed patent on this technology. So it's patent pending and it's going to be available to our, all our customers.
Chris: That's exciting. That is exciting.
Chris: Like I've, when you start thinking about the time to value, like you said, but just, in reality, the time it takes to actually do these matches and things like that, now it's hours instead of weeks or months. Wow. Okay.
Sushant: Keep going. Yeah. What else? Yeah. Another one I would like to highlight out of the many is relative intelligent assistant, right?
Sushant: As you might have heard, right? Generative AI is how powerful it is to write to simplify customer experiences, right? So customers can tap into the power of generative AI, which are these aided by large language models in the background to new levels of impact for their team members, right?
Sushant: Relative intelligent assistant or RIA as we call it. It unlocks a new experience for users to use natural languages, query relative for [00:21:00] finding the right information insights with real, we can amplify business users, data stewards, or any persona, we can amplify the impact by eliminating hours of manually searching profiles using natural language to find relevant information, right?
Sushant: Whether users are looking for information of, particular terminology on how to do a particular task, for example, how to merge two profiles and relative, or actually doing data exploration to create dynamic segments, right? You can actually write natural language queries, talk to Ria or talk to relative or show me all profiles in a particular region for that particular speciality create any complex query.
Sushant: And Rhea will be able to understand it and present you the data that you are looking for, right? So data exploration particularly becomes really easy and simple with this, right? Gone are the days where you would set up advanced queries that would take five minutes to set up and then give you the results, right?
Sushant: You're using Rhea. You can just talk [00:22:00] to like you're talking to a friend and then get all the data.
Chris: Yeah, Rhea's kind of this. You guys take it. Reltio takes it a step further, but basically Rhea is you type in a question and it gives you an answer, but whenever it's embedded into the product, then it can do that.
Chris: Plus, there's this one example I saw that you can get different graphs or something like that. Can you talk a little bit about that? Because that's a really cool feature that I've never seen before, besides with royalty, I'm not saying it's not happening, but for me, it was like, Oh my gosh, this is on a if a customer wanted to do it on their data, it's able to do that kind of stuff.
Sushant: No, of course. So as I said, in the beginning it's very important to understand the context of customer data. And then respond based on that. So what radio customers can now do is interact with Ria to get insights about the data that they already have in relative. So they could make, ask your hey, how many, organizations in a particular industry like [00:23:00] retail are are my customers and let's say New York, right? And then you will find that information show, right? Or they can say, Hey, give me a bar chart of all my customers by industries.
Sushant: And Ria will not only find those customers by industry, but generate a bar chart on the fly, which customers can then add to their regular dashboard, or they can just slip it and take it away for, whatever meeting they're headed to. Multiple different applications, right? You can query across, I would say.
Sushant: Objects are across entities, right? Search based on their location, search based on their any kind of attribute or any kind of property that, that is stored on the entity or on the profile, Rhea can search through it and derive insights. I
Chris: love it, man. We could probably talk about Rio a whole entire podcast, but we have to get to the next thing, but this is so exciting.
Chris: And probably this next question, I'm going to be extremely excited to hear what you say, but, can't wait to see what's coming. So what enhancements [00:24:00] involving AI and ML are planned for Reltio's product and what can our customers expect?
Sushant: Yeah, we have a very exciting road map, for infusing across our platform.
Sushant: We call it applied AI. So we're going to invest in key three key areas, right? First of his augmented entity resolution to drive, 10 times the data store productivity. We want to reduce dependency on any manual configuration. Of rules for entity resolution or matching and augment data stewards for tools to get their jobs done faster, right?
Sushant: I spoke about fern That's just the first step and we will continue enhancing and keep improving their These models and these models are going to be available for every single domain out there, right? persons organizations supplier product, going across industries healthcare licenses anything like that, right?
Sushant: They will be models, right? So for every industry, every domain, we are going to have models that will help customers. The second part is [00:25:00] improving time to value by applying or infusing AI in ingestion and data modeling, right? We will introduce capabilities like automatically understanding the schema of customers data, right?
Sushant: Right now, customers spend a lot of time doing data modeling. We are, we plan to introduce capabilities where we can just read the data and then create the data model within Realtio automatically. So that reduces a lot of configuration time to bring a new data sources into Realtio. And when you are ready to load that data into Realtio, ingest that data into Realtio, we are going to provide capabilities that automatically map Customer data to the relative data model, right?
Sushant: So that is the next level of automation that the IML will bring again to reduce time to value. So the idea here is that, to get the business outcomes from the relative that customers need, it should not take them weeks. It should take them days. And we are going to get there by using ML machine learning.[00:26:00]
Sushant: Wow. And third one is data governance, right? Data quality. I would say that is an extremely important thing, right? We spoke earlier, how important is data quality across the enterprise to make any AI initiative successful, right? So we are. Adding capabilities within relative like anomaly detection, right?
Sushant: Data is constantly coming into the radio from 2020, 30 sources in a typical enterprise, right? We can now, create time series data and continuously measure data quality, right? And flag any anomalies based on historic references, right? So if your data quality metric, like fail rate was 60, 70%.
Sushant: Over the last six months, but suddenly you bring data from a new source. And the fill rate drops to 40%. We will flag it as an anomaly because our machine learning models have learned it through it. And then data stewards will have, alerts and recommendations on how to fix that immediately.
Sushant: So that is another area that we're going to [00:27:00] invest in on flagging anomalies, giving recommendations to data stewards and making suggestions how to improve data quality. So these are the, some of the areas that, that we are. Going to invest within our roadmap over the next months and quarters.
Chris: I love that. And if I'm wrong, just tell me, but as I think of time to value, it feels like Realtio is making the implementation of a data unification platform, like Realtio easier, quicker, is that kind of the thinking behind some of that as well, or, Oh, yes. We
Sushant: already, yeah.
Sushant: We already started with our journey last year when we introduced. Velocity packs, right? But that, that took some effort out of that, but whatever time is left, there are a lot of opportunities for bringing efficiencies for our customers, right? And AIML is, I would say, is Enables those tools for automation, right?
Sushant: I think that's the natural way to go.
Chris: Got it. Wow. [00:28:00] That's really exciting. So just a couple more questions. How important is it for businesses to have a clear AI strategy and vision? So we're thinking future out, but, and what should it include, what should be included in an AI robust strategy?
Sushant: Yeah, I think that's a great question, right? I think personally that the most critical strategy even before any AI strategy is the data strategy. Data strategy comes before any AI strategy. Customers should develop a comprehensive data strategy that addresses data collection, storage, governance, quality, security, and privacy consideration, identifying data sources, Establishing standards and policies, ensuring accessibility and reusability of data, reusability for interoperability is extremely important for AI initiatives.
Sushant: That is very important, right? And we're, that's where products have a major role to play. That's what should define a clear vision for AI [00:29:00] adoption and articulate specific measurable goals and objectives aligned with business priorities. I believe there is, there was a time when AI was, or more of it was an experiment, but the kind of investments customers are doing, they have to be aligned to what kind of outcomes that you are expecting out of your after a few investments, right?
Sushant: Whether you want to improve efficiencies, grow revenue, it has to align with something, right? Revenue growth, cost reduction, customer satisfaction customer experience, competitor differentiation. Align it. Otherwise it is very hard to justify the investment, right? The customers also need to assess and invest the necessary technology, infrastructure, tools, and platforms to support AI development and deployment, right?
Sushant: We have seen there's so many offerings all across the board, right? Whether customers want to do it themselves or go to a hyperscaler or Niche players just doing AI ML, right? We spoke about earlier, right? Should we do it in house or or do use off the shelf? That's a key [00:30:00] consideration that customers should consider in their AI strategy, right?
Sushant: One of the key things also that that is challenging at this moment is availability of right talent and skills, right? Building a skilled workforce with expertise in AI, including data science, machine learning, software engineering, and domain knowledge is going to be very critical, right? So they need to start recruitment, start investing within the talent that is already there in their company, and generally develop a culture of AI literacy, I would say, right?
Sushant: Yeah, I would say one, one more thing that is really important is, considering ethical and responsible AI. We spoke about earlier about biases, right? We have to be really responsible on how we are looking at customer data in particular, right? Making sure we, we invest the right technology and resources to have security privacy built in.
Sushant: We are not letting bias creep in into our [00:31:00] models. And we always have a human centric design with full transparency and accountability so that, your initiatives are well respected and accepted across the enterprise as well. That is really important. And yeah, these are some of the things that I believe are going to be key in developing a robust AI strategy and customers need to consider while building some strategy.
Chris: Yeah, it's good. So last question for you Sushant. So what challenges do businesses face when scaling AI solutions across their operations and how can they address some of these challenges effectively? I think you might've hit on some of this stuff already.
Sushant: Yeah, I think we did covered but Yeah, maybe I can take a couple of examples here that I'd say the biggest one that we see every single day when we talk to our customers is, data silos and integration issues, right?
Sushant: Many businesses struggle with data fragmented, fragmentation across different departments and systems, right? One of the biggest problems we see is use case specific. [00:32:00] within customer, they set up their own data systems, and then it is generating data, which is duplicate and not of a good quality.
Sushant: And you can't simply run the initiative there, right? You need high quality data. So that's where data unification platforms are built. can help customers unify fragmented data from across the enterprise and provide trusted real time data for AI initiatives. Real to use data products like Customer 360, they enable customers to deploy this interoperable data, again, across the enterprise for business responsiveness, right?
Sushant: So very important to remove these data silos. And introduce a concept of data product ownership so that it is all data is always reusable or interoperable across the enterprise. The second is lack of standardization, right? Inconsistent data formats and processes across departments again can create roadblocks when integrating AI.
Sushant: Solutions into existing workflows, right? Everybody's generating customer data [00:33:00] in different formats and nobody's following any standards, right? So I would say, if you look at principles like data products and data mesh, right? Customers should develop standardized data formats or data contracts, metrics and processes to streamline this integration across the departments.
Sushant: And something again, I alluded earlier, right? Skills development and talent acquisition, right? This might become right. A big challenge, right? So the expertise. required to build, maintain, and manage a solution can be scarce, right? This is my struggle to find or retain data scientists, engineers, and AI specialists needed for large scale adoption.
Sushant: And we, I think we all read on how difficult it was to find at a certain point, data scientists in Bay Area itself, right? Need to like, Plan for it, right? Companies need to, business need to invest in skill development, whether it in house or looking for skills outside training program and talent acquisition initiatives to build a skilled workforce with expertise in [00:34:00] AI to prepare for the future.
Sushant: I think that's going to be key. So these are some of the, I would say challenges and how these challenges can be addressed effectively by my customers.
Chris: Sushant, wow, that was some great insights about what Reltio is doing from an AI ML. Great
insights just from your thought leadership on, the future outlook of AI and how businesses should think about it.
Chris: I really appreciate you coming on, Sushant, so thank you so much.
Sushant: Oh, it was a great discussion, Chris. Those were some really insightful questions. Thanks for having me here.
Chris: Yeah, of course we'll do it again. And so thank you everyone for tuning in to another data driven podcast. I'm Chris Detzel. Don't forget to rate and review us.
Chris: Thank you everyone.