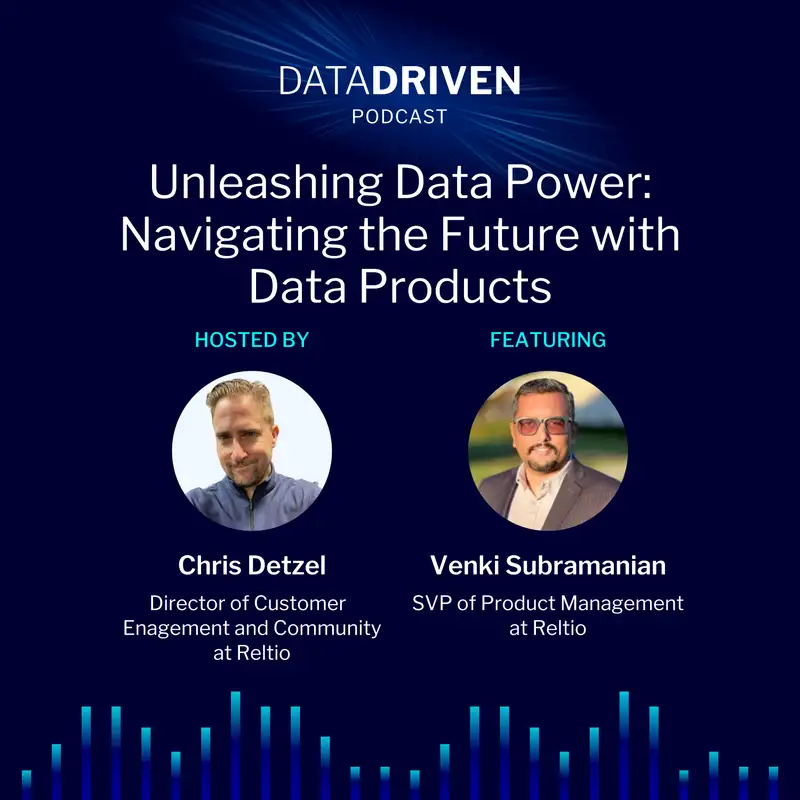
Unleashing Data Power: Navigating the Future with Data Products
Chris: [00:00:00] All right, welcome to another data driven podcast. My name is chris detzel and today we have special guest Venki supermanian From reltio and he is the senior vp of product Venki. How are
Venki: you today? I'm doing very well chris. How are you?
Chris: I'm doing well, man. I'm really excited
Venki: to have you on I'm excited
Chris: to be here.
Chris: Good. So one of the things that we're going to dive deep into is the future with data
Venki: products. What do you think? That's great topic. Very relevant. So let's talk more about it.
Chris: All right. Before we do, why don't you give us a little bit of background about yourself, who you are and
Venki: those things.
Venki: Absolutely. I'm happy to do that. Like you said, currently, I'm the Senior Vice President of Product Management at Reltio. I have been here four years now seeing the company go through an exciting growth phase during my time here, working with a lot of strategic customers across industries.
Venki: Helping them with their data infrastructure, with [00:01:00] especially their, core data products. So that's why this topic is very near and dear to my heart. Prior to coming to Reltio, my experience has primarily been in the enterprise software SaaS space. More on the application side, focusing on customer experience, CX, CRM, applications across companies like SAP and ServiceNow.
Venki: And in all of those places Chris, I think we have talked about it before as well. I've told you that. I've seen the challenge that customers go through in realizing the value from all their investments in digital transformation, customer experience transformation and things like that.
Venki: Even though they have the best software, probably the, the most modern software and technologies available to them because of data holding them back, right? Yeah. So that's what really attracted me, into the space of data management and, here I am four years later at Reltio, and I'm really excited to be talking to our customers and partners and collectively solving the problem of data and making companies use data as a strategic asset.
Venki: Yeah,
Chris: and [00:02:00] I, it's such an interesting field, to be honest, and I've been with Reltio for three years doing I would say exactly what you do, but learning about data, master data management, data unification and, data products, which we will focus in on today. Now, before we dive in specifically on data products, there's some somewhat of a confusion in the market about.
Chris: Data products, data as a product. Can you just briefly talk about some of the differences between those and maybe some similarities real
Venki: quick? Absolutely. So data as a product was a concept that actually came into prominence through data mesh. Data match as a, principle of data management was popularized by the mark and several others in the industry and it is gaining popularity, right?
Venki: When I have conversations and Chris, probably you share the same experience as well. We see our customers use more and more of the data mesh principles and how they are organizing their teams, how they approach data and data governance and data unification kind of capabilities. So data as a product really [00:03:00] came out of that.
Venki: And there are some key characteristics of what constitutes treating data as a product, right? The first and foremost is to really not to build new data sets for every single requirement that comes up in an organization. And really look at how do we create some reusable capabilities as we assemble data together.
Venki: And when those kind of data sets are created, there are four things that I would highlight that came out of the data as a product. Approach. One is the discoverability of data so that people within the organization who need to use data or operate on data, they have an easy way to understand or discover the data sets of the, the data that is being curated within an organization.
Venki: So that's one of the fundamental principles of data as a product, the data sets that are curated in that manner needs to be addressable so that people can understand what is there, where is it coming from, what is the meaning of the data, if you think of something like a. a Go live date for a customer as an example.
Venki: For digital software like ours, that is something we hear very [00:04:00] often, but the interpretation might vary depending on who you ask. So in the data, when you treat data as a product, it is important to have consistent definition and address ability of the different data elements.
Venki: That is another key part, the very important aspect of treating data as a product is interoperability so that you are creating a single unified view of the relevant data that can then be. Connected to various downstream systems in various modes, right? Real time applications versus operational systems versus analytics and things like that.
Venki: And in a interoperable manner, which means that you're not trying to create again, different copies of the same data for different consumption purposes and all that. And the same data can be consumed in different fashion. And last but not least that it is trustworthy and secure, which means it has characteristics like data quality and security and governance kind of aspects built into it.
Venki: So this is broadly. The data mesh definition of treating data as a product data product,[00:05:00] again, I don't want us to get caught up in the definition here, right? That's not. That's not the right way to approach this because ultimately we have to look at what are the capabilities needed.
Venki: Data products are specific products that are created out of the data that augments the data with insights and make it actionable. And there are various examples that we can see for that, right? Simplest example would be the customer segmentation data product that is created that is used by marketing to activate customer segments, specific customer segments, right?
Venki: That includes the raw customer data. It could include certain algorithms that are attached to it. That might predict the buyer propensity. It might have augmented segmentation capabilities that are added to it. So think of the customer 360 or customer data set for marketing as a data product that actually has the right data for a specific set of consumption scenarios.
Venki: So a lot of similarities, I would say the similarities are more than the differences even in the word itself, data as a product. And it's confusing, but I think my approach is [00:06:00] always to look at. What is needed as opposed to getting caught up in the technicalities of the definition? Yeah, and I appreciate that
Chris: Venki.
Chris: There's lots of kind of these types of words that are You know put out there like data mesh data fabric data as a product data product and a bunch of other things like that So it's good to get a view of what you think and things like that. So I appreciate that as you look at Reltio customers, for example, using data products.
Chris: Do you see see that happening today? Any customers
Venki: doing that? Absolutely. Several customers, that are already creating or curating their data and calling them data products and approaching it as a data product. And when I say approaching it as a data product, if you do. If you look at the similarities between any product management, digital product management, the discipline that I have been in most of my career, right?
Venki: Yeah. 1 of the key aspect of a digital product, think of a product our CRM system, for example, right? Every company uses their CRM [00:07:00] systems with probably some unique capabilities or processes that they implement. So the customization or configurability of a base product to suit different scenarios, to fit to different scenarios is one of the key characteristics of a digital product.
Venki: And you can apply the same concept to a data product as well, right? So when companies bring together the data sets, they are looking at not creating. use case specific data products, but use case agnostic data products can be applied to a common set of use cases in a configurable manner. The same example that I just talked about for, the customer data set for marketing without a lot of changes could also be used for white space mapping and maybe sales execution, right?
Venki: That is really what makes it a data product as opposed to creating unique data sets. So one aspect is configurability for Different users for different use cases from the same core capability that you create, which is how we approach digital products and how we should approach data products as well.
Venki: The 2nd characteristic is the [00:08:00] ability to deliver iteratively regular product enhancements. So you may not have a product or a version of the product that meets everybody's every single requirement, but you have a road map to actually. Enhance the base product that you deliver over time and add more capabilities to it.
Venki: And that's exactly how many of our customers are approaching their data products, right? When they start with their implementation of relative, um, instead of solving or creating this. amazingly wonderful capability that is going to be everything for everybody. They are looking at how do I deliver my first value within the first 30 days?
Venki: How do I activate most consumer or customer of this data, right? The internal stakeholder, how do I make that person or that team successful with their first set of use cases? And then I go on to do the same thing 30 days later, the next 30 days later, right? So we are looking at iterative value delivery, which is a.
Venki: a characteristic, a typical feature of any digital product and how any product manager would approach it. Similarly, our customers have data product owners or data [00:09:00] product managers who are applying the same principles on their data and looking at iterative value delivery with the shortest time to value to start with.
Venki: The third aspect, another key aspect is reusability of existing process and machinery instead of creating new bespoke processes or approaches every single time, right? If you look at product management as a discipline in the digital product world, we have common practices of how do we collect. Inputs requirements.
Venki: How do we ideate? How do we create definition and scope and then how do we turn that into execution and then delivery and then not, it doesn't stop there, right? Digital products. Also, product managers are responsible for driving adoption, so they go all the way across the life cycle with the same consistent approach and they're able to deliver different products or different capabilities within the same product.
Venki: Apply the same exact principles to data products. www. microsoft. com What we see our customers do, they are starting off with really understanding their customer base. The internal [00:10:00] stakeholders are even external. If the data product needs to be exposed externally, they look at the core set of capabilities or data in this case.
Venki: With augmented insights and other kind of things with the metadata definitions, the discoverability aspects, all of that, right? They're defining that and they're applying the same exact principle to create newer versions iteratively on the same data products or even new data products, that consistency of approach using or reusing the existing machinery as opposed to every data team approaching their data set differently and creating different kind of capabilities.
Venki: Is a fundamental shift that we are seeing in data teams and, the data engineering teams today. So three things that I would highlight how we see our customers approach it. The configurability aspects is creating the same data product with enabling multiple use cases, the iterative delivery and reusing of the same set of processes and the same way of engaging with stakeholders all the way to driving adoption of the data products that have been [00:11:00] created.
Venki: I can share, a number of great examples of, some of our customers doing it, but this will be principles.
Chris: So I appreciate those principles. I think that's a good start. And so I was thinking about that. I was thinking let's say somebody implements Reltio and we start looking at kind of this data, product kind of thinking, can you walk me through a Hey we use it, we use relTO, but then a customer, whatever that, example is using data as a product in a real life example and how others might reuse some of that stuff.
Chris: I don't know if that makes sense, but I want a real live example of and how you might really
Venki: use that. So if I were to think of a real life example we can look at a customer like a car max. We have a public case study. I believe of this video and they are actually consolidating their customer data, customer 360 and the vehicle data, right?
Venki: We all know what business car max is in. [00:12:00] And their core goal is to get a customer in and out of their lot with a successful sale in the shortest time possible. And that results in improving customer satisfaction. It results in improving operational efficiency. And obviously it results in growth additional revenue and growth for CarMax.
Venki: The two key domains of data that they focus on are the customers and vehicles. At any point, they want to see the full 360 degree view of the vehicle. A vehicle would have probably gone through multiple sales within CarMax itself. You bought it first, you sold it back to them, maybe three years later, then they sell it to me.
Venki: I use it for the next, maybe five years and I sell it back and those kinds of things. So they want to understand the full history because that's an important aspect of what their customers need and what they need internally, right? Similarly, they want to know a customer who might have multiple interactions across digital channels or their, in person interactions where I walk into a CarMax lot and I have a conversation with a sales representative and all that.
Venki: So they not only want to track the customers. Basic sort of demographic [00:13:00] or, those kind of attributes. Like they obviously want to know who I am, where do I live and my address, my contact details and things like that. But they also want to know about potentially my income level, education level, various other determinants of kind of purchase might I do my propensity to buy.
Venki: And things like that, right? So a lot of that information actually needs to come together. And you can think of those two as fundamentally two different data products that they've created the customer data product and the vehicle data product customer 360 vehicle 360, right? And it didn't, it includes every piece of interaction as well that they might think as relevant, right?
Venki: So they might want to know how many times have I actually contacted in the last three months, because if they see repeated contracts or contacts or inquiries from me about certain types of vehicle. Obviously, that indicates my propensity to buy a certain thing. It indicates that I'm actually in the market and things like that.
Venki: So you can think of augmenting the raw data with some of the calculated insights and things like that. So that could be one practical example of how a customer is [00:14:00] using Reltio for a specific, couple of data domains and treating that as a product with multiple ways in which it can be used. Now, that was one more interesting thing, Chris.
Venki: I told you usually it is not a single use of the data. So in case of CarMax. They're also tracking the interactions that their sales teams might have. The team members might have with a specific person and a vehicle that results in a sale because they actually want to use that information for their sales compensation.
Venki: Because if you and I both talk to a. A customer potential customer, and then I was a person finally closed the sale. We both have probably a share of that sale, right? In terms of competition that is allotted to us. So that is another kind of another use case. They're able to enable through this information with the customer vehicle, the sale and the interactions associated with that.
Venki: to actually then determine how do they compensate their sales team members. So that is an example of how you essentially look at the same data, but you enable multiple applications or use cases on top of the [00:15:00] same data, which is a characteristic of a data product. Now that was, thank
Chris: you. And that's exactly what I was trying to get at in a real life example.
Chris: And CarMax is a great example of that. What are some of the, what's the biggest challenge or problem that you think that Data product solve. I think you mentioned a little bit of that, but just want to go a little bit deeper into that question.
Venki: Yeah, I believe the fundamental problem that it solves is 1 of aligning the data related investments to business value, right?
Venki: Directly aligning it to value and shortening the time to value by focusing on iterative value delivery as opposed to. The old words are a waterfall approach of, I'll develop everything and then I will do a big unveiling and then everyone is going to be super excited about it, right?
Venki: That's not the recipe for success. We know that. So those are the two fundamental problems and. If I may just touch upon or drill down into each one of them for just a little bit, the first one, traditional way of [00:16:00] how think of a data warehouse that companies stood up, right? They would start with, I have this magical single repository of all my data, you call it a data lake, data warehouse, whatever, and you start pumping all your data into it and very soon it becomes a mess.
Venki: You have no idea where, what kind of data is being brought in. You have no idea who is going to consume the data. And if I'm a data consumer and I'm looking for a specific kind of data, I am still struggling to figure out where it is, what is it. And how do I use it? And it typically goes back to an I. T.
Venki: Or a data engineering team who will need to then help me go through that every step of the way. So if you turn that around and say that these data products now provide visibility into what kind of data is there, what kind of quality is there? And you focus on that decentralized governance or ownership and things like that.
Venki: And there is a data product owner is Who is then also responsible for not just creating the product, but also driving the adoption of the product. Now you're starting to align the kind of data that you bring together and the [00:17:00] use cases or the applications of the data that you enable, uh, very tightly from the beginning, right?
Venki: So you don't start with let me bring all the data and then I'll figure out how to use it. You're starting from the exact opposite end of the spectrum where you say. What kind of data do you need, right? And how do I get that together? And I can go and talk to three, four different stakeholders. And I, as a data product owner will be responsible for creating the commonalities.
Venki: Understanding the commonalities and then bringing the rights data to my data product platform, whatever it might be. So we are flipping the approach completely. And that again results in driving adoption, driving value. Otherwise, it becomes a technology investment in almost a solution waiting for a problem.
Venki: Whereas with data product approach, we are flipping it in the right manner, really starting with the problem and then working back from there to identifying or creating the right solution. So that is one. And then the other one I mentioned was you may not satisfy every single use case and every single stakeholder on day one, but by focusing on the iterative delivery, you're again able to [00:18:00] shorten the time to value and really drive meaningful adoption of the capabilities and really tying your investments.
Venki: Two outcomes.
Chris: I love that. And I think that's so important. That gets me to the, this question here. I think it's even more important. What are some of the challenges and measuring the value of data products? And how do you think some organizations success or successfully able to
Venki: do that? I would say the first, probably the most important and the fundamental challenge in measuring value is.
Venki: The fact that it becomes it is usually an afterthought and not something that is that they start with. My previous example of how that's right. Traditionally, maybe we are talking about 10 years back, 5 years back, depending on the company's maturity, um, they look at. Solving a technology problem and then figuring out what is the business application or relevance of what is, the capabilities we are creating becomes an afterthought.
Venki: And then maybe several months [00:19:00] down the years down the line, you're questioning the value. What did I get from all that in this right now? So that is fundamentally the problem, I think, which like we talked about data products are solving by starting really with the consumers and their needs.
Venki: And then, starting with how do I solve that specific need? So take an example. If we are creating a data product. For customer specifically focused on my marketing efficiency use cases, the KPIs might be increased conversion rates for the campaigns that I run, right? Reduce time for conversions, right?
Venki: So faster conversions and things like that. So there could be a few key metrics, which means I need to improve my segmentation capabilities so that I'm targeting the right kind of customers with the right campaigns. And if that happens, then obviously your conversion rates will go up. The time to convert will also go down.
Venki: Those could be KPIs that are related to the data product, right? And you can think of those as the ultimate business outcomes. You can work back from there and then determine or define what are the data [00:20:00] product specific KPIs that could then translate to freshness of the data. How quickly are you able to get the data from its sources to the consumer side of the application so that I can actually run a campaign.
Venki: Targeted at a specific segment within an hour of the data becoming available, right? As in, if you show up as a new prospect on our website, can we target you back within an hour or within even minutes, right? Can you get immediately a notification from us? Or can we then start personalizing the content that you see, which actually looks at the freshness of the data?
Venki: You might have data quality characteristics that are incorporated into it so that we don't get the segment wrong for a specific customer. So we can start from the end business outcome and work back into more specific data product related success criteria. And we can measure it that all along the way.
Venki: But it has to start with business outcomes first. Absolutely.
Chris: Are there any tips that you'd give to organizations seeking to value its data
Venki: assets?[00:21:00] Tips for valuing the data assets? I would simply say this Chris in today's world, right? Data is one of the most important assets that any company has, right?
Venki: So I don't usually get questions anymore about, how do I value my data? Definitely there are questions every day that we talk about, we discuss around how do I articulate the value or how do you, how do I correlate the value that I'm delivering with the kind of investments that I'm making in my data infrastructure or, data governance layer.
Venki: But, um, yeah. It's less a question about how to value my data. But I think there are still a couple of considerations that companies should have in that area. One is, when you think of data products the ultimate business consumer or the business outcome that you're trying to deliver obviously has a value associated with it.
Venki: So that'll be one easy way to look at it. And that could also result in prioritization of your investments that you're making. So not every data product has the same [00:22:00] priority within an organization at any given time. It's just like any other investment that we make. So how do you prioritize the right investments based on the outcomes, the ROI of the investments that you're going to make?
Venki: And that orientation of starting with end outcomes is something that is fundamental to data products. That's one. The second aspect is. Many companies have start are now starting to also monetize their data products. I was actually talking to one of the leading financial institutions who actually sell various different products across.
Venki: Wealth management asset management investment bank, various different things. And I actually met a VP of data products there and this person's sole responsibility, her sole responsibility is really to create those data products that are then sold, monetized and sold. To their end customers, because those customers want visibility into the ROI that they're getting from the services that leveraging they're leveraging from this company.
Venki: So I'm working with this bank or [00:23:00] by this financial institution. And over time, I've done several transactions and, I'm leveraging different services. These data products essentially provide single consolidated view of various aspects of my services or products that I'm leveraging, and it can show value and it can also probably augment the kind of services that this company might be able to offer, right?
Venki: So that is the other aspect that I'm starting to see now where we've been talking about data monetization for several years. I don't think it is a very new concept, but again, the approach of data is really products. That can unlock multiple value scenarios is starting to actually make it a reality more and more.
Chris: And I thought, when I first asked that question, you weren't going to, you're like, ah, yeah, I don't know, but that was good. That was really good. How would an organization begin to create kind of a data product and You know who are really the key people involved in what technology and talent is needed.
Chris: That's a big question
Venki: big few questions Yeah, that's a great question, right? Because so [00:24:00] far in our conversation because we've been talking about how wonderful it is
Chris: Yeah, how wonderful it is, but now We need to challenge and how do
Venki: you do it? Exactly. Let's talk about the execution aspects. Like, how do you make it a reality?
Venki: So first and foremost, I think this needs to start with really a mindset change and change in an approach. And to some extent, I believe it is top down, right? We have to really start at the top, create that buy in and create that kind of vision. From the very top of the organization. Sometimes it's the CEO himself or herself or the CDO or, whoever is responsible for data strategy within a company and they need to set the the bar high and say that, we have to get these kind of outcomes and approaches.
Venki: This is outcomes from the data. Hence, we are going to approach it as a product. And then, you go down from there. One of the key roles in any data product organization is the role of a data product owner. This data product owner is the glue between the technology or the IT side of the house and the business side of the house, right?
Venki: That connective tissue is extremely important. Because you know very well [00:25:00] that they always don't speak each other's language. That's right. Business is looking at driving business outcomes and they have specific needs, but they when they express the needs, those things need to be translated into what are the technology investments of the data related investments that need to be made, which the data teams, the data engineering or the it teams understand.
Venki: So the data product owner is one of the key. Roles in that. And before you keep
Chris: going on, I would say I Manishh and I spoke to Joe DeSantos a few a week or two ago about that particular role. And he said that role almost doesn't exist. It does, but it's a very hard to find because it's a way newer type of role.
Chris: So it's not easy to find those people. So you might have to kinda teach, learn, teach, learn kind of stuff there, right? Because there's not a lot of
Venki: those folks out there. That is so true. That is so true, Chris. And that is why I think, we talked a little bit about digital product management principles apply to data.
Venki: You really need to find someone who understands the product [00:26:00] management principles, but it's flexible enough to apply those things into a new area new domain, essentially to the domain of data, right? And this person needs to be obviously very, strong with their business acumen to understand and have conversations with the business stakeholders.
Venki: There's a lot of prioritization discussions they need to have. So they need to be very influential and so there are personality traits as well that we will need to look at for a person who's sits at the intersection of data technology and business. But that is exactly and you're right.
Venki: Multiple people like Joe that we have talked to, they've all acknowledged the need for this, but also said how difficult it is to find the right person. Yeah. But, if you look at the other key roles I would just simplify it and say that, you need a strong business representative or multiple business representatives who can be your stakeholders because you're essentially driving adoption of the data products within that community.
Venki: So it is really important to have a strong participation in the partnership. And not have them come into the picture almost at the end of, creating a data product [00:27:00] and then expect them to adopt whatever you have developed. Equally important are the technology representatives. A data architect who can actually come in, collaborate, be a partner with that data product owner in creating the right set of capabilities in a repeatable reusable manner because you don't want to create every data product as a completely bespoke or a completely new thing, right?
Venki: You don't want to start with a clean slate every single time. So it is important to also have that architect's point of view who can create the consistency and bring the right kind of technical capabilities and help the product owner create. Or bring that data product vision come to life.
Venki: Is there any specific
Chris: technologies that that you think is needed to do some of this stuff, or is it just your basic, data
Venki: technology tools? If you think of one of the fundamental aspects of our data product it is you're bringing data from multiple sources, some of it, first, first party sources, like your own data from different systems, [00:28:00] augmenting it with the right kind of external data sets, third party data sets.
Venki: And creating this unified interoperable view of the data. So one of the fundamental technologies that I believe that is an enabler for data product is a data unification platform, right? And I'm not saying this selfishly because I'm here at Reltio and I see it every day. I truly believe that is the foundation because if you were to draw out the picture of a data product on a piece of paper, you will essentially have multiple sources feeding into this centralized view of the data and then multiple consumers connected to the data, right?
Venki: So that, that hub in the middle that collects all these disparate data sets from different places and then makes it a data product with multiple consumption scenarios is essential. A foundational technology of this is a data unification platform like a Reltio that can actually create the data product to be the foundation for your data product.
Venki: The other two areas or two or three things that I would highlight is one for a data product really to go from just a consolidated data set to a data product. You need to [00:29:00] also look at the augmented capabilities that you need to provide around data consumption. How do you make sure that there are different types of consumption points?
Venki: There, there is a term data contract. That is actually used more and more to represent the aspects of how data gets published and how it can be consumed and the various consumption metrics. So the part that actually enables the multiple consumption is an important aspect. The data discoverability is another part, right?
Venki: So metadata capabilities that actually describe what kind of data sets in the data product and the lineage of where the data comes from and gets consumed and all that is another important aspect. These are not necessarily different products. I think a customer would be more successful in looking at a platform that can enable multiple those multiple of those capabilities as a single integrated platform like what relative provides, but that is foundational to the success of a data product from a technology point of view.
Venki: And the last piece is the expectation is that [00:30:00] the data products are augmented with insights or derived, aspects, right? So you can think of the data products falling into multiple categories of raw data, augmented data algorithms. Insights and visualizations and things like that. It can have all of those aspects.
Venki: So another key aspect is can the data product or what kind of technology is needed to actually augment the data product with the predictions with AI machine learning technology be brought to the data as opposed to the data being taken to them. You have to bring AI to the data and then create augmented insights so that the consumer of the data actually gets more value than just Consolidated view of the raw data itself.
Venki: So that will be another key point that I would highlight, which should be from a technology or a capability point of view. Part of a data product platform. Yeah, you didn't go into
Chris: detail yet about my last question, but I've got two more questions for you. I think and one is. When you look at data product, is that somewhat of a new [00:31:00] relative term?
Chris: And where do you see that going in the future? Maybe there's specific use cases that you envision or things like that.
Venki: Yeah, I would say it is relatively new as a term, right? We talked at the beginning of the podcast about how data mesh constructs refer to the term data as a product. Data product has been used on and off, before, but it's relatively new.
Venki: But. The concepts are so powerful and the value of, approaching data in that manner is so evident to many of the companies that the adoption is happening really fast. I'm encouraged to see more and more of our own customers that we talk to on a daily basis, Chris, really adopting not just the terminology, but really the essence of what it provides and really approaching data in that manner.
Venki: So data product can happen while it is new, I would say the adoption is happening really fast. I, where I see the augmentation happening, what, what is going to actually make this richer than where it is today is really the aspects [00:32:00] around, um, more of the, make making the third party data and enrichment capabilities easier so that you're not just relying on the data that sits in the, within the boundaries of a single enterprise.
Venki: But you're augmenting the data in meaningful ways from third party data sets and that consumption of the third party data sets that are relevant to each of the domains is going to I would say it's going to be made easier with the technology with the advancements that we are making. If you look at the broader ecosystem companies like Snowflake AWS.
Venki: Google Azure, they're all investing in a lot of the data exchange or data marketplace capabilities where they are curating these data sets and making it easy for you to combine that with your own data. So that is one of the aspects that I see where we are going to see. Faster innovation and more capabilities.
Venki: The other one which we not talk about is the power of AI, right? AI is going to fundamentally augment every aspect of how data products are created and they're consumed. Technology wise, you might [00:33:00] be probably waiting to ask me this as we see a subsequent question.
Chris: That's right.
Venki: I'm jumping ahead because, we cannot have a conversation about data without talking about AI, right?
Venki: There are two aspects that I would highlight. One is how do you apply AI machine learning capabilities to augment the creation of data to improve the discoverability of the data? To improve the data unification capabilities and to also improve the consumption. The second aspect is applying AI to derive insights that are not evident, not most obvious in the data itself, right?
Venki: Going back to the example that we talked about at the beginning, like customer data for marketing use cases. Companies are already using AI for determining customer lifetime value, customer churn score, propensity to buy, various other aspects, which are key to targeting the right customers with the right message and and giving them the service or the product that they expect, right?
Venki: That those capabilities are going to further improve. And [00:34:00] further get simplified. They have existed for a while. Large companies with large budgets, with large data science and machine learning teams have been able to do it. But I would, I think we're going to see a democratization of those technologies where it's going to be available for everybody, irrespective of their size, irrespective of the amount of, budget that they have.
Venki: Technology is going to get simplified dramatically, and that's going to augment the whole world of data products and infuse that intelligence. No doubt.
Chris: I think you answered my last question around, the impact of AI and ML and the role it plays in data products. Is there anything I missed that you're just, that you're like, you should ask this question?
Venki: I don't think so. But I think, that AI part, even though we saved it to the end, I think it is an important aspect Chris. And. I'm glad that we touched upon it and I said, we could not have not talked about it in a conversation like this. The, generative AI and chat GPT and those kinds of things are the talk of the town, right?
Venki: Yeah. There is an interesting blog that Sanjeev Mohan had [00:35:00] published recently it's available on medium. And Sanjeev talks about how you could even look at chat GPT as a data product, right? If you look at it, what is it? It's basically a collection of data in that specific case. It's text data that actually processed in a specific manner with a product that is then get that got created out of it, which will essentially answer your questions by using these.
Venki: Large language models, right? Generative transforms. So
Chris: yeah, man, it'll cost you 20 bucks to get the that particular product is going to cost like Claude to is another example of. Basically the same thing, right? So they are creating products out of the data.
Venki: Yeah, absolutely. And that, so it's an interesting analogy and I had not thought about it before, like ChatGPT as a data product, but Sanjeev explains it beautifully.
Venki: And we AI is going to change the way we unify data, right? Relative has experiments that we are running with [00:36:00] LLMs, large language models used to identify the same version of two records in two different versions, maybe even across languages. If you were to travel to, let's say Germany and they wrote down Chris, Chris, Christopher Detzel in German the language wise is probably the same, right?
Venki: So maybe I'll use another example. If you were to go to Dubai and they wrote it down in Arabic or something, how can it compare Chris Detzel, Christopher Detzel, C. Detzel and a fourth version of that is probably written in Arabic. And identify that you are the same exact person, because if we are working with international companies with global operations think of a hotel chain that operates across multiple companies, including places, Dubai and Saudi Arabia, they have a need to unify the data and AI is going to actually simplify those kind of capabilities dramatically.
Venki: Provide capabilities that we did not have maybe three, five years back. And I think
Chris: B2B companies like ours it's key for us to dive in as quickly as possible so that we can differentiate ourselves, and I think that's everybody's. [00:37:00] Running towards that. So it's not just us, but it is so important.
Chris: Venki, thank you so much for coming on the data driven podcast. And next time we'll, this should be out in the next few weeks. So please rate and review us. And Venki, thanks again for coming. Appreciate
Venki: it. Absolutely. It's my pleasure. Great talking to you, Chris, and talking about the topic that we had today.
Venki: Looking forward to it. We will
Chris: do more. Take care. Thanks.
Creators and Guests
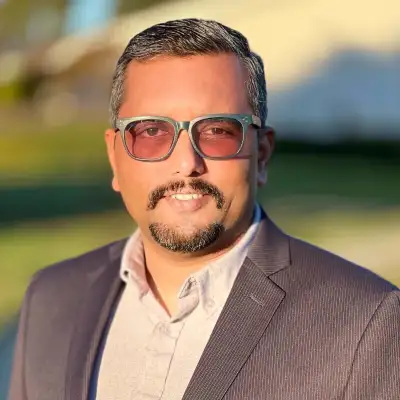